
Extract meaningful information from unstructured data
Break down data barriers with Nanonets AI—extract valuable information from documents, emails, tickets or databases. Transform unstructured data across multiple sources into actionable insights.
Automate complex business processes with AI
Automate even the most complex manual workflows with Nanonets' no-code platform. Our learnable decision engines equip your team with the tools they need to make faster, more informed decisions.
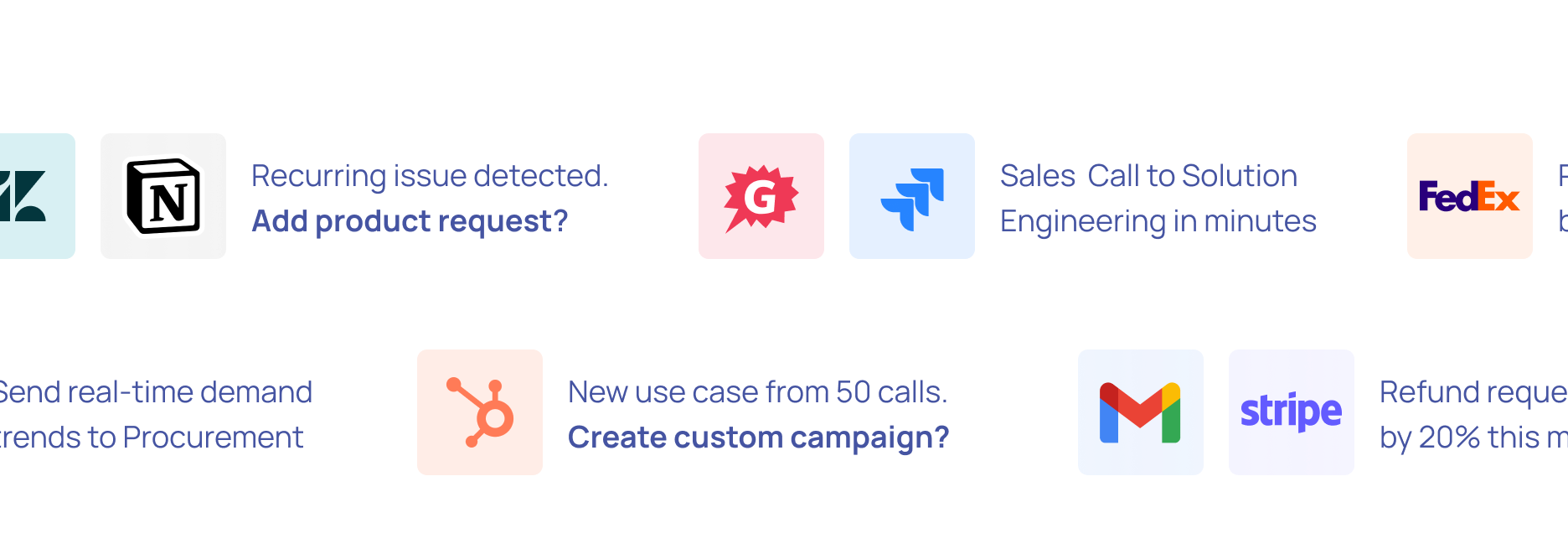