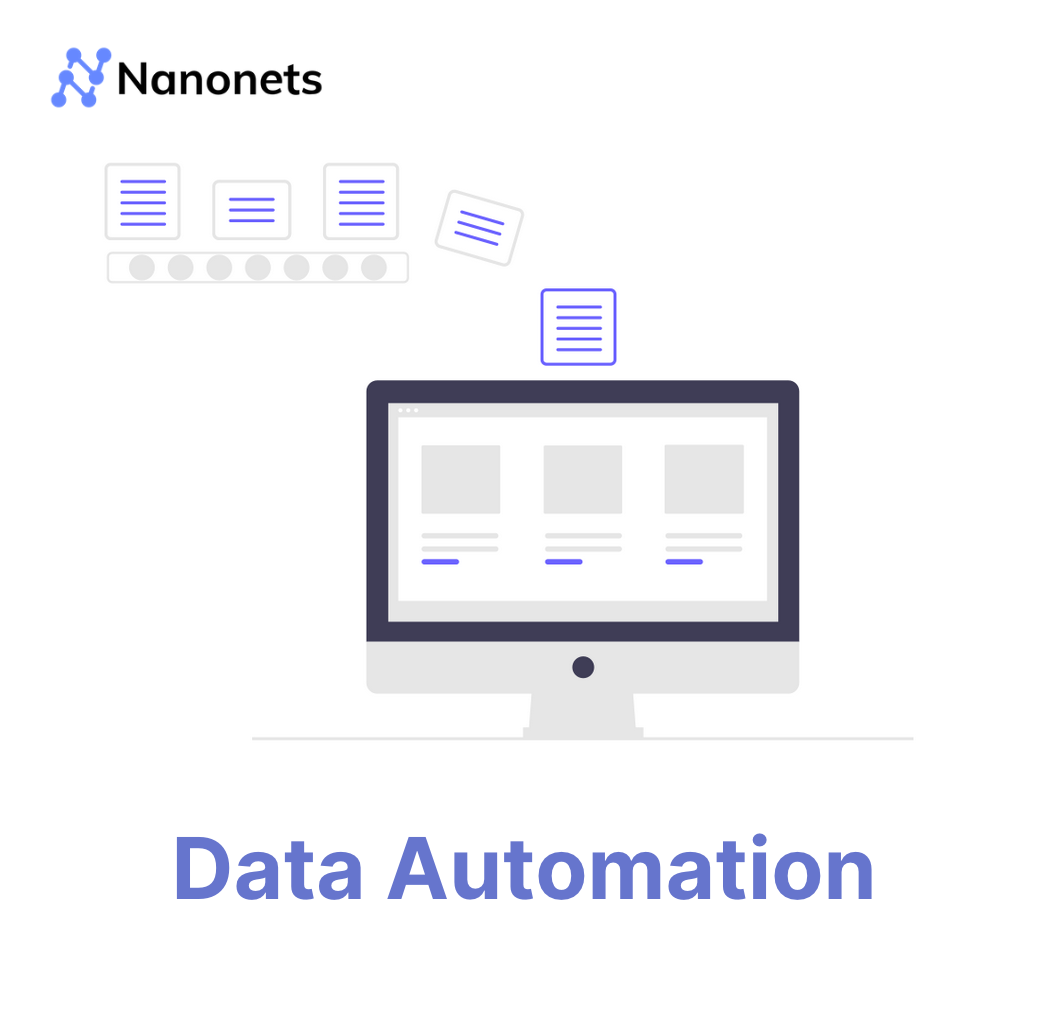
With the increasing amount of business data, data automation has become critical. It is a transformative solution to streamline data workflows, reduce manual intervention, and improve overall data management.
This guide will look at data automation, components, benefits, various use cases, and how automation can streamline it.
What is data automation?
Data automation, facilitated by data processing systems, entails the automated uploading, handling, and processing of data, replacing manual intervention.
It involves three essential elements: Extract, Transform, and Load (ETL).
It uses technology, specifically artificial intelligence (AI) and machine learning (ML), to automate data collection, processing, and management.
Data automation uses intelligent algorithms and software tools to optimize data-related activities. By automating these processes, businesses can reduce the time and effort required to handle large volumes of data, improve data quality, and gain insights.
It is a critical tool for organizations to stay competitive and agile in the digital age. According to Mordor Intelligence, the data center automation market is expected to grow at a CAGR of 17.83% from 2018 to 2028.
What are the components of data automation?
Data automation includes the following main components:
- Data extraction is the process of collecting data from various sources, such as documents, websites, databases, and emails. AI-powered data extraction tools can intelligently read and interpret unstructured data, extracting valuable information for further processing.
- Data transformation is the process of converting the extracted data into a standardized format and structuring it for easy analysis and integration with other systems.
- Data loading is the process of loading the transformed data into a target system, such as a data warehouse or a business intelligence platform. Automated data loading ensures that data is updated in real time and readily available for analysis.
- Data integration is the process of combining data from multiple sources to create a unified and comprehensive view of the data. Data automation facilitates seamless data integration, allowing organizations to break down data silos and improve data accessibility.
- Data quality management is the process of ensuring data accuracy, completeness, and consistency. Data automation tools can help identify and rectify data quality issues, enhancing the reliability of data for decision-making.
How does data automation work?
Data automation typically follows steps; every organization may need to add, remove, or modify some to suit their needs. Below are some of the essential steps to how data automation works:
- Data collection: This involves collecting data from various sources, such as databases, spreadsheets, documents, and websites.
- Data integration: This involves combining data from different sources into a single repository.
- Data cleaning: This involves removing duplicate records, correcting errors, and standardizing data formats.
- Data processing and analysis: This involves using algorithms or machine learning models to extract insights from the data.
- Data visualization and reporting: This involves creating visualizations and reports to communicate the insights from the data.
- Data storage and management: This involves storing the data securely and making it accessible for future use.
- Data integration with business processes: This involves integrating the data automation solution with the organization's business processes.
- Data governance and compliance: This involves ensuring the data is handled according to industry regulations and internal policies.
Data automation is a complex process, but it can be gratifying. By automating your data workflows, you can improve the efficiency of your operations, reduce costs, and make better decisions.
What are the types of data automation?
Data automation encompasses various types of automation solutions that cater to different aspects of data management and processing. Some of the key types are:
Data Integration Automation: This type of automation integrates data from different sources and formats into a centralized database or data warehouse. It involves tools and platforms that facilitate seamless data integration, ensuring that data from various systems can be accessed and analyzed together.
Data Cleaning Automation: Data cleaning is a crucial step in data management, as it ensures that the data is accurate, consistent, and error-free. Data cleaning automation tools use algorithms and rules to identify and correct errors, remove duplicate records, and standardize data formats.
Data Transformation Automation: Data often needs to be transformed into a different format or structure to be used effectively. Data transformation automation involves tools that can convert data from one format to another, such as unstructured text to structured data.
Data Processing Automation: This type of automation focuses on automating data processing tasks, such as aggregating and summarizing data, running calculations, and performing statistical analyses. It enables organizations to process large volumes of data quickly and accurately.
Data Visualization Automation: Data visualization automation involves tools that automatically generate visualizations based on processed data, such as charts and graphs. These visualizations make it easier to understand complex data and identify patterns and trends.
Data Reporting Automation: Data reporting automation automates the generation of reports based on processed data. These reports can be customized to meet specific requirements and automatically sent to stakeholders on a scheduled basis.
Data Monitoring and Alerting Automation: This type of automation involves setting up automated alerts and notifications based on predefined thresholds or conditions. It helps organizations proactively monitor data and identify potential issues or anomalies.
Machine Learning and AI Automation: Machine learning and AI automation use advanced algorithms and models to analyze data, make predictions, and generate insights. These technologies enable data automation systems to learn from data and improve over time continuously.
Robotic Process Automation (RPA): RPA involves using software robots to automate repetitive and rule-based data entry, extraction, and processing tasks. RPA can significantly reduce manual effort and improve data accuracy.
Data Governance Automation: Data governance automation focuses on automating data governance policies and rules enforcement. It ensures that data is handled and used in compliance with regulatory requirements and internal policies.
Combining these different types of data automation solutions provides organizations with a comprehensive and efficient approach to data management and processing. By automating various aspects of data handling, organizations can optimize their data workflows and leverage data more effectively for decision-making and strategic planning.
What are the benefits of data automation?
Here are some of the key benefits of data automation:
- Improved accuracy: Data automation can help to reduce human errors in data entry and processing. This can lead to more accurate insights and decisions.
- Increased productivity: Data automation can help streamline data workflows, freeing employees to focus on more strategic tasks.
- Better decision-making: Data automation can help organizations make more informed decisions by providing real-time insights into their data.
- Cost savings: Data automation can help organizations save money by reducing the need for manual data entry and processing.
- Improved data security: Data automation can help organizations protect their data by implementing security measures such as encryption and access controls.
- Better data governance: Data automation can help organizations improve the quality and consistency of their data by implementing standardized processes and procedures.
- Enhanced customer experience: Data automation can help organizations deliver personalized and relevant customer experiences by giving them more profound insights into customer behavior.
- Competitive advantage: Data automation can help organizations gain a competitive advantage by giving them access to real-time insights and making faster and more informed decisions.
What are the limitations of data automation?
It is important to note that data automation is not a magic bullet. It has its limitations, which include:
- Data quality: Data automation is only as good as the data it uses. If the data is inaccurate or incomplete, the automation results will also be incorrect or incomplete.
- Complexity: Data automation can be a complex process, and it can be challenging to get it right. There are many factors to consider, such as the type of data, the complexity of the processes, and the availability of resources.
- Cost: Data automation can be expensive, especially if it is done on a large scale. The cost of the tools and technologies, as well as the cost of implementation and maintenance, can be significant.
- Change management: Data automation can also disrupt existing processes and procedures. It is essential to manage change carefully to ensure that the transition to automation is smooth and successful.
- Human oversight: Data automation can never wholly replace human leadership. There will always be cases where human intervention is necessary, such as when automation encounters unexpected data or a need to make subjective decisions.
Best Practices for data automation
Here are some best practices for data automation:
- Start with a clear understanding of your goals and objectives. What do you hope to achieve by automating your data workflows?
- Identify the suitable data sources and processes to automate. Not all data is created equal, and not all processes are worth automating.
- Choose the right tools and technologies. Various data automation tools are available, so it is essential to choose the ones that are right for your specific needs.
- Get buy-in from stakeholders. Data automation is a team effort, so getting buy-in from all stakeholders, including business leaders, IT staff, and data analysts, is important.
- Implement the solution carefully and monitor its performance. Data automation is a complex process, so it is important to implement the solution carefully and monitor its implementation to ensure it meets your expectations.
- Test and validate the automation solution. Testing the automation solution thoroughly is important to ensure it works correctly.
- Document the automation solution. It is important to document the automation solution to maintain and update it in the future.
- Monitor and update the automation solution. It is important to monitor and update the automation solution as needed to ensure it meets your needs.
By following these best practices, you can ensure your data automation project succeeds.
How do you choose the right data automation tool?
Selecting the appropriate data automation tool involves carefully assessing several vital factors. First, consider your specific data automation needs and objectives, ensuring the tool aligns with the processes you aim to streamline.
Evaluate the tool's compatibility with your existing systems and data sources, as seamless integration is vital. Look for user-friendly interfaces and intuitive functionalities that match your team's skill level.
Scalability is another crucial aspect; opt for a tool to accommodate your organization's growing data demands. Prioritize tools that offer robust security features to safeguard sensitive data. Lastly, consider the tool's support, training resources, and community engagement to ensure effective implementation and utilization.
Nanonets is a powerful AI-based platform that empowers businesses to harness the full potential of data automation. With its advanced data extraction and processing capabilities, Nanonets can revolutionize how organizations handle data, driving efficiency, accuracy, and innovation.
Here are some of the benefits of using Nanonets as a data automation tool:
- Nanonets excels in processing unstructured data, such as invoices, receipts, contracts, and forms. Organizations can gain valuable insights and make data-driven decisions by automating the transformation of unstructured data into structured formats.
- Nanonets offers seamless integration with existing systems and workflows, making it easy to implement and use. Its user-friendly interface allows organizations to create custom data extraction models tailored to their unique requirements.
- Coupled with robust data security and compliance, Nanonets can safeguard sensitive information. The platform follows industry-standard security protocols and ensures data processing adheres to regulatory requirements, giving businesses peace of mind.
- Nanonets offer scalability, enabling organizations to handle large volumes of data efficiently and effectively. Nanonets can quickly adapt as business needs evolve to meet changing requirements, providing a flexible and future-proof solution.
If you are looking for a robust and reliable data automation tool, Nanonets is an excellent option. With its advanced capabilities and features, Nanonets can help you automate your data workflows, improve data accuracy, and gain valuable insights.
FAQs
What is data automation?
Data automation is the use of technology to automate data-related tasks.
Why is data automation important?
Data automation is important because it can help organizations to save time and money, improve accuracy, and make better decisions.
What is an example of data automation?
An example of data automation is using a software program to automatically generate reports from a database.