What is Data Extraction? (Definition, Types & Challenges)
What is Data Extraction?
Data extraction is the process of acquiring and processing raw data of various forms and types to improve the operational paradigms of an organization.
It is perhaps the most important operation of the Extract/Transform/Load (ETL) process because it is the foundation for critical analyses and decision making processes that are vital to organizations.
It enables consolidation, analysis and refining of data so that it can be converted into meaningful information that can be stored for further use and manipulation. The extracted data can help in decision making, customer base expansion, service improvements, predicting sales and optimizing costs, among other things. Data extraction can thus help improve productivity and safeguard a company’s core competency.
With data becoming the lifeblood for businesses worldwide, exracting meaningful data is a vital operation that defines the line between success and failure. Not surprisingly, the global data extraction market that was valued at $2.14 billion in 2019 is projected to reach $4.90 billion by 2027.
Data Extraction Example
Consider a retail business utilizing data extraction to analyze customer purchase histories. By extracting information on popular products and buying patterns from sales records, the company can optimize inventory management, strategize marketing campaigns, and enhance customer satisfaction based on tailored recommendations and promotions. This exemplifies how data extraction empowers businesses to derive actionable insights from large datasets, fostering informed decision-making and operational efficiency.
Why is data extraction needed?
“I cannot build bricks without clay,” said Sherlock Holmes. Data is the clay to the brick of business operations.
Data extraction offers the means to glean valuable insights from a myriad of textual sources. The importance of extracting data stems from its capacity to distill voluminous and complex information into accessible formats that cater to various needs.
Texts that are information-dense and lengthy can be challenging to comprehend fully. Data extraction enables the extraction of key information, allowing for quicker understanding and decision-making. This holds true not only for textual data but also for content disseminated across the internet in formats like PDFs, web pages, word documents, and more.
Furthermore, data extraction breaks language barriers by facilitating the translation of texts published in unfamiliar languages. This empowers individuals to access and understand information that might otherwise remain inaccessible due to linguistic differences.
Businesses stand to gain significantly from data extraction due to its potential to harness diverse data formats. By extracting data, businesses can leverage this information for a multitude of purposes, including marketing campaigns, research initiatives, and strategic decision-making. The acquisition of data isn't solely about accumulation but rather about the insightful application of information.
Data-driven decisions are among the most compelling reasons for businesses to invest in data collection. The ability to analyze collected data assists companies in making informed decisions promptly. This decisiveness proves crucial in a fast-paced business environment, enabling businesses to adapt swiftly to changing circumstances and capitalize on opportunities.
Moreover, data enhances customer satisfaction by enabling personalized experiences. By studying the effects of their efforts on customer satisfaction, businesses can identify areas for improvement and tailor their offerings to meet individual preferences. In turn, this drives customer loyalty and referrals, positively impacting sales and brand reputation.
Data isn't just a passive asset; it actively contributes to revenue and profit growth. By scrutinizing data, businesses can optimize operations, identify profitable actions, and pinpoint areas for expense reduction. This financial acumen ultimately leads to increased revenue and improved profitability.
Data is also a potent tool for solving complex problems. Its extraction and analysis allow company leaders to identify and address critical issues systematically, enabling them to monitor the outcomes of proposed solutions. Data-driven insights help improve company processes, uncovering inefficiencies and optimizing operations.
Data extraction versus data mining
Data mining and data extraction are terms frequently used interchangeably in the realm of data science. They are not the same. Data mining extends beyond the scope of just pulling the data, encompassing a more intricate array of activities.
Data extraction is the foundational step that kickstarts the journey towards data utilization. It involves the methodical collection of raw data from varied sources, enabling consolidation into a central repository. While data extraction serves as the precursor to data mining, its focus lies on gathering and centralizing data without necessarily uncovering patterns or insights. This collected data is then prepared for subsequent processing and analysis, laying the groundwork for informed decision-making.
Data mining transcends mere information retrieval, and deals with analysis, insights, patterns, and relationships within a dataset. This process involves employing advanced algorithms and techniques to analyze substantial data volumes, discerning correlations, predicting future trends, and extracting invaluable knowledge. Data mining's aim is to uncover previously undiscovered information, providing organizations with the ability to make informed decisions and gain a competitive edge.
Types of Data
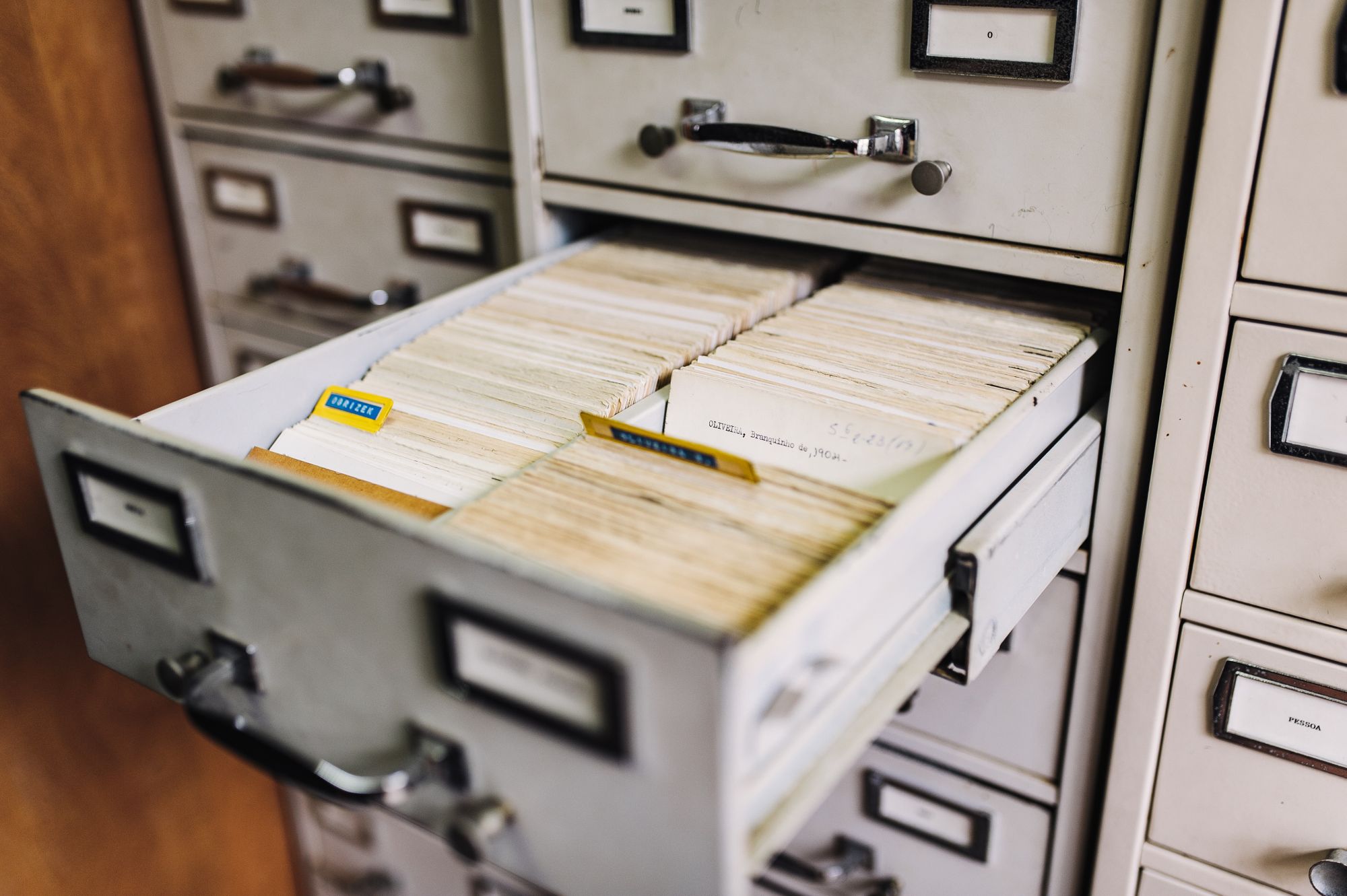
Data may be classified according to their source:
- Physical sources: Physical sources of data may include one or more of the following - books, journals, magazines, newspapers, brochures, marketing materials, paper-invoices, paper-POs and letters. The extraction of data from these physical sources is typically manual and strenuous since it involves the efforts of human beings to look into the source, extract the data and input it into the destination. These days, simple digital tools such as OCR – optical character recognition – scanners can lighten some of the burden of data extraction from physical sources. Most scanners these days have OCR functions built in, to convert printed characters into digital text.
- Digital Sources: Data may be present in digital sources such as word processing files, digital spreadsheets, webpages, e-invoices, digital bills, emails, and online and offline databases. Data scraping or web scraping are activities that can extract relevant data from these digital sources.
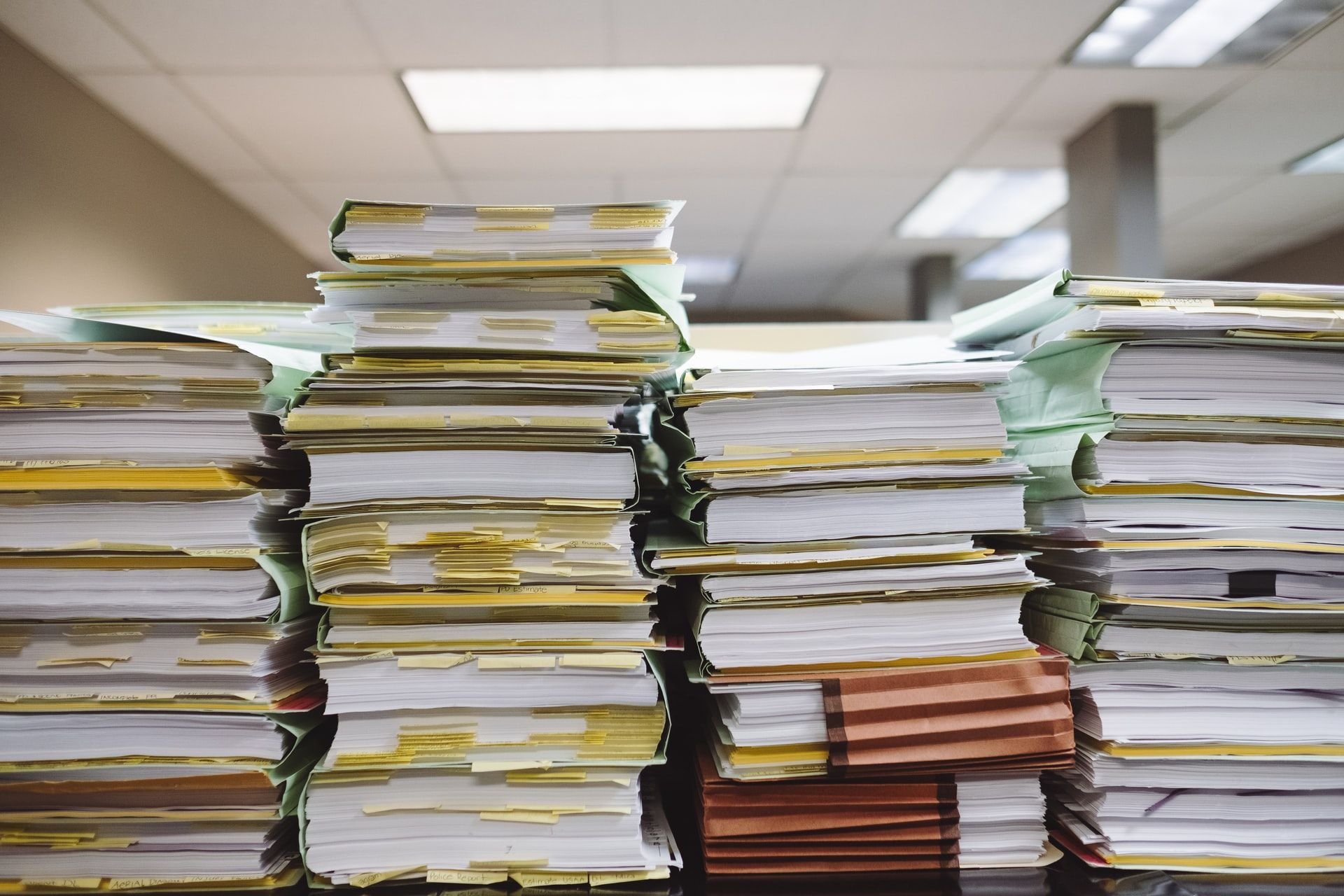
Data are also classified based on their structure at the source:
- Structured Data: When the data source already has a logical structure, it becomes convenient for extraction. An example is the extraction of phone numbers from a digital directory which is already organized based on a logical scheme. Data that is stored in a structured format such as a relational database management system (RDBMS) is easy to extract using tools such as Structured Query Language (SQL). Tools such as SQL can also perform some amount of T (Translate) and L (Load) operations from the ETL system that makes it a particularly powerful tool.
- Unstructured Data: This is the form in which most data exist – as disorganized or unorganized bits of information that must be judiciously sifted and sieved for sensible extraction of data. The sources of unstructured data could be web pages, editable documents, PDFs, emails, scanned text, spool files etc.
Data extraction from unstructured sources is performed by one of three ways,
- Using text pattern matching to identify small or large-scale structure
- Using a table-based approach to identify common sections, for example using a standard set of commonly used headings; and
- Using text analytics to understand the context of the data.
Finally, data may be classified according to its nature:
- Customer Data: Most service and product providers have a customer database that includes their names, phone numbers, email addresses, identification numbers, and purchase history, and in case of online businesses, their social media activity and web searches.
- Financial data: These are for accounting processes and include information on transactions, such as sales numbers, cost/price, operating margins and even some amount of competitor information. These types of data help monitor performance, improve efficiencies, and make strategic decisions.
- Performance Data: This is a broad category and could include data related to tasks or operations, such as patient outcomes in the healthcare setting, sales logistics for a trading company, etc.
Want to scrape data from PDF documents, convert PDF to Google Docs or convert PDF to Excel? Check out Nanonets PDF scraper or PDF parser to scrape PDF data or parse PDFs at scale!
Data Extraction for ETL
Extracting Data is a pivotal component of the ETL process. It entails the systematic retrieval of data from various sources such as databases, spreadsheets, digital invoices, APIs, and logs. This initial phase serves as a precursor to subsequent transformation and loading stages, collectively facilitating the conversion of raw data into actionable insights.
The significance of data extraction is underscored by its influence on the overall effectiveness and integrity of the ensuing data processing pipeline. The techniques employed in data extraction determine the quality and relevance of data that is subjected to subsequent transformation and analysis.
Data is found in a spectrum that spans structured to unstructured forms. Structured data follows standardized models, making it ready for analysis. Logical data extraction is the common method for extracting structured data and is categorized into full and incremental extraction.
- Full Extraction: This method entails retrieving all data from the source without considering changes or modifications. Comparable to a comprehensive survey, this approach guarantees the incorporation of all available information from the source.
- Incremental Extraction: In this technique, solely the data that has undergone changes since a specific point in time is extracted. Comparable to a targeted review, this method hones in on recent modifications to minimize redundancy and expedite processing.
Extracting unstructured data is more complex due to the diverse types of data sources, such as web pages, emails, PDFs, and more. Although complex, unstructured data is valuable as a source of actionable information and requires processing beyond simple extraction, Preparing unstructured data for analysis needs further work, like removing whitespace, symbols, and duplicates, and filling in missing values - a process often called pre-processing.
How does Data Extraction work?
Extraction tools fall into three main categories, each catering to specific needs:
- Batch Processing Tools: These tools help in transferring of bulk data between locations. These are useful in the extraction of data from legacy and outdated sources. Such tools are best suited for in-office data management.
- Open Source Tools: These are small-scale data extraction tools that are either budget friendly, or even free and best suited for small, budget conscious organizations and small-scale operations.
- Cloud-Based Tools: Most commercial data extraction tools are cloud based and have advanced functionalities that can extract data from a variety of sturctured, semi-struictured and unstructured sources. They often use AI functionalities for discerned data extraction and have features that assist compliance, minimize delays and enhance data security.
In all of these cases, the data extraction process involves the following common steps:
- Uploading the Document: Physical documents are uploaded into a digital system through scanning. Attachments are saved into appropriate folders.
- Image-to-Text Conversion: Optical Character Recognition (OCR) technology is employed to convert the digitized document content into a plain text (TXT) format, although still unstructured.
- Parsing to Structured Format: A parser processes the TXT file, structuring it into a more organized format such as JSON, XML, XLSX, or CSV. This structured data is then easily processed and analyzed.
- Optional Verification: Extracted data can be cross-referenced with third-party sources for validation and compliance.
Advanced data extraction tools implement third-party APIs for extracting information to streamline the process across various industries, including finance, retail, accounting, customs, and healthcare. These APIs provide cost-effective and efficient solutions for integrating data extraction into existing software systems, eliminating the need for complex in-house development.
Modern tools have AI features that allow for intelligent data extraction
Data Extraction without ETL
While it is possible to have data extraction tools that are not part of ETL, such stand-alone systems have a few limitations. Extracting raw data without transforming or loading it properly can result in raw unstructured data, which may be difficult to analyze and use in other software systems. While this type of data might be okay for keeping records, it might not be very useful for much else.
A transition to automated data extraction is always better when it is part of the ETL process. This ensures that the data isn't simply digitized but is also transformed into a form that can be easily processed in subsequent manual or automated processes.
Another downside of standalone extracting data is that it can be slow and inefficient. Most stand-alone data extraction tools require some level of coding, which is time-consuming and requires a certain level of coding expertise.
Comprehensive ETL systems provide valuable benefits: they enable seamless migration of data from external sources into company-wide databases and consolidate different data types from various systems into one place. This enhances efficiency, simplifies sharing data with external partners while keeping control, and enhance accuracy by reducing the likelihood of errors from manual data entry, editing, or re-entry. This not only maintains data integrity but also minimizes time spent on fixing errors.
Types of Data Extraction
There are two types of data extraction techniques:
1. Logical
This type of extraction is again of two sub-types:
- Full extraction: All data is extracted at the same time, directly from the source without need for additional logical/technological information. It is used when data must be extracted and loaded for the first time. This extraction reflects the current data available in the source system.
- Incremental extraction: The changes in source data are tracked since the last successful extraction given by the time stamp, and the changes are incrementally extracted and loaded.
2. Physical Extraction
When source systems have certain restrictions or limitations such as being outdated, logical extraction is impossible and data can only be extracted by Physical Extractions. There are two kinds of physical extractions:
- Online Extraction: There is direct data capture from the source system to the warehouse. This entails direct connection between the source system and the final repository. The extracted data is more structured than the source data.
- Offline Extraction: Data extraction takes place outside the source system. The data in such processes can either be structured by itself, or structured via extraction routines.
Data Extraction Tools
Data Extraction tools are software that automatically extract data from the source. A good tool will be capable of extracting data off of a variety of sources such as forms, websites, emails, and more. Such tools are used by businesses to generate leads, extract information from public documents and webpages of competitors, identify trends, and improve the analysis of otherwise unstructured information.
Data extraction software may be integrated with data quality software and data preparation software to clean and organize data after scraping. It can also be combined with data integration software so that multiple data types and sources can be aggregated in one place. To qualify for inclusion in the Data Extraction category, a product must be able to:
- Extract structured, poorly structured, and unstructured data.
- Pull data from multiple sources.
- Export extracted data in multiple readable formats.
There are three kinds of tools that are used for data extraction:
- Batch processing tools extract data in batches.
- Open source tools are useful with limited budget and provides basic services that may be sufficient for small companies
- Cloud-based tools focus on streaming extraction of data as part of the ETL The capture is done as and when data becomes available and processed right after, which eliminates any time delays that can be caused by batch processes.
Advantages of Automated Data Extraction
The advantages of automated data extraction include:
- Improvement of accuracy and reduction of human errors: Automation can eliminate many of the human errors that are brought about by oversight or fatigue.
- Time savings: Automation is undoubtedly faster than manual extraction of data. Time is often money in businesses and a moment saved could be a moment earned in monetary terms.
- Freedom from repetitive tasks: Freeing the employee from mundane data extraction tasks can enable use of their skills for more productive activities. This can improve employee morale and the company bottomline.
- Better control and access to data: A centralized location of structured data makes it more accessible to all stakeholders and participants in the business, thereby allowing coherence in business activities.
- Cost benefits: While the initial investment on automation can be daunting, the cost savings through productivity improvements, employment morale and time savings can more than make up for the setting up costs of automated data extraction systems.
- Scalability: Automated data extraction systems offer scope for scaling up of the business without worrying about the volumes of data that would correspondingly be scaled.
Nanonets has interesting use cases and unique customer success stories. Find out how Nanonets can power cognitive data capture for your business.
Challenges to Data Extraction
The most common challenges to data extraction processes, especially when it is a part of the ETL system are:
- Coherence of data extracted from various sources, especially if the sources are both structured and unstructured. AI based data extraction tools can be trained to collate data in a sensible manner that make them suitable for post processing operations.
- Data security is another area that can be challenging in data extraction applications. Financial data, for example, are highly sensitive and data security must be ensured by organizations that use automated data entry tools for data management.
Many data entry tools like Nanonets, come with a robust technical assistance team that can help overcome the challenges and harness the full potential of automated data entry operations.
Extract Data from documents using Nanonets
Nanonets is an ideal choice for data extraction within the ETL process due to its AI-powered Optical Character Recognition (OCR) tools that are tailored for intelligent document processingLeveraging advanced OCR, machine learning, and deep learning techniques, Nanonets effectively extracts relevant information from unstructured data. This solution is characterized by its speed, accuracy, user-friendliness, and the ability to craft custom OCR models from scratch, complemented by seamless integration with Zapier.
The following features of Nanonets make it an ideal part of ETL automation:
- Nanonets eliminates the need for manual pre-processing of poorly scanned documents or varied formats. Its automatic pre-processing adapts to alignments, fonts, and image quality, streamlining the entire process.
- Output can be fine-tuned and exported into various formats like CSV, Excel Sheets, and Google Sheets, which enables further data analysis and processing.
- Nanonets offers pre-installed installations with platforms like Zapier and UiPath.
- Nanonets allows users to construct models for custom data, catering to noisy images while ensuring results are delivered with heightened accuracy and speed.
Nanonets can intelligently extract data from a range of sources including,
- Number Plates: Employed for traffic regulations, parking management, and security enhancement in public spaces.
- Legal Documents: Facilitating digitization, database creation, and searchability for various legal forms like affidavits and judgments.
- Table Extraction: Automatically identifying tables in documents, extracting text, and column headings for research and data entry.
- Banking and financial documents: Analyzing cheques, passbooks, KYC compliance, loan applications, and account management.
- Menu Digitization: Extracting menu information for food delivery apps like Swiggy and Zomato.
- Healthcare: Digitizing medical records for easier access and searchability by doctors.
- Invoices: Automating data extraction from bills, receipts, and invoices for retail and logistics industries.
Nanonets' efficacy is validated by tangible benefits reported by its users. Customers have achieved remarkable outcomes, including an 80% reduction in accounting costs and a 3-5 times return on investment within a 3-month payback period. Success stories like Expatrio's 95% reduction in manual data entry time and Advantage Marketing's fivefold business scale through Nanonets automation underscore its real-world impact.
If you handle invoices, receipts, or any other document that must be digitized and processed for other business operations, click on the link below to know more about Nanonets' Data Entry Automation Solution.
Nanonets' intelligent document processing use cases help organisations adopt automation seamlessly. Here are some interesting case studies:
- Expatrio uses Nanonets to save 95% of time spent on manual data entry
- Advantage Marketing scales its business 5x times using Nanonets automation
Update June 2021: this post was originally published in June 2021 and has since been updated.
Here's a slide summarizing the findings in this article. Here's an alternate version of this post.