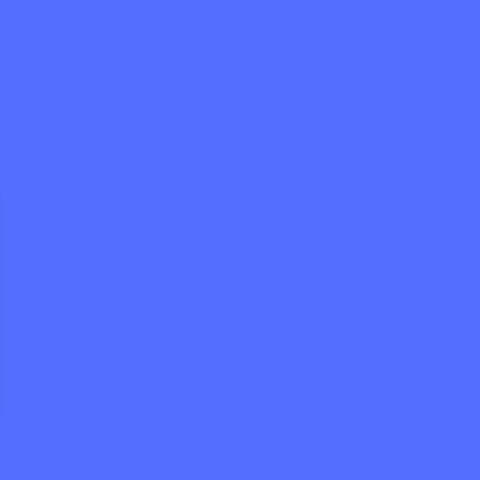
Introduction
This blog post will take us through how a business which need to ensure customer due diligence (CDD) can automate the KYC (Know Your Customer) processes using deep learning and computer vision based solutions. But before we get started, let’s familiarise ourselves with some basic terminology.
Customer Due Diligence - CDD involves verifying that your customers are who they say they are and assessing the risks associated with each customer like the possibilities of fraud, money laundering, terrorism financing, etc. This includes verifying your customer’s name, address, photograph by analysing bank documents, utility bills, etc.
Anti Money Laundering - AML refers to a set of laws, regulations and procedures meant to prevent criminals from disguising illegally obtained assets and funds as legitimate income by safeguarding against trading illegal goods, tax evasion, market manipulation, corruption of public funds, etc.
Know Your Business - KYB involves vetting a business trying to establish a relationship with a bank by determining their Ultimate Beneficial Owners (UBO) and enforcing compliance by assessing risks associated with the business. You can learn more about beneficial ownership structures and a risk-based approach to counter laundering here.
Know Your Customer - KYC involves the procedures required to enforce CDD and AML directives by acquiring and verifying a customer’s identity and the associated risks of doing a transaction with said customer.
After mishaps and scandals like the Danske scandal or the Panama Papers leak, understanding the vulnerabilities of an organization to fraud and laundering-related crimes and implementing compliance mechanisms is taking precedence.
Leverage the power of AI and automate the KYC process with Nanonets Intelligent Automation Software!
Who needs KYC?
The types of businesses and professionals who fall under the ‘regulated sector’, that is entities that need to comply with the Anti Money Laundering directives as per their jurisdictions need a robust KYC procedure for onboarding and timely reviews.
These include
- Banks and credit institutions
- E-payments, e-currency services
- Bookmakers and casinos
- Adult websites
- Legal professionals
- Estate agents
- Accountants and tax advisors
The professions and business types that fall under this regulated sector are steadily increasing. To find out more about the international standards regarding AML, check out the 5th EU AML Directives here, the FATF AML guidelines here and the FinCEN guidelines here.
This report lists the things you should make sure to consider when approaching a managed solution provider for KYC and AML compliance services.
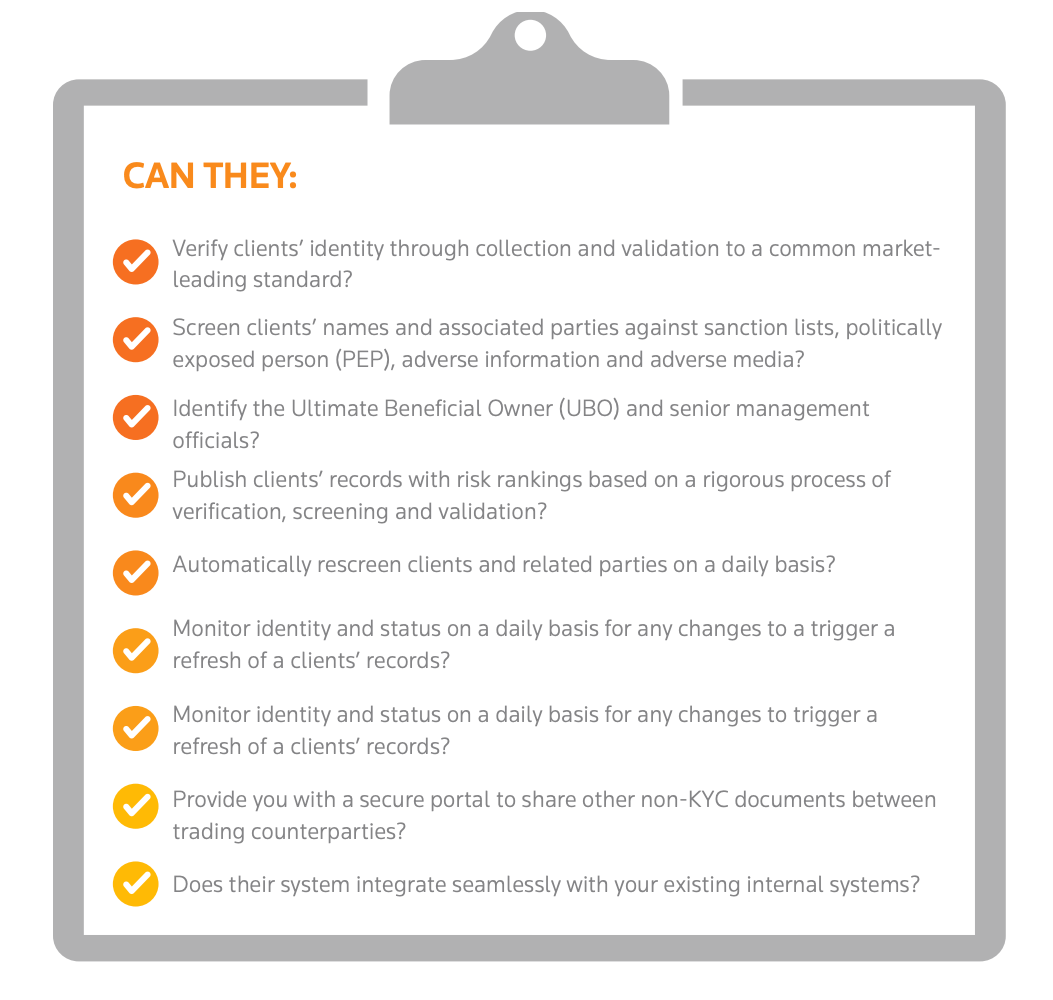
KYC process currently
The KYC process for a customer requires an ID proof and proof of address. For an ID proof, the customer can present documents like Passport, Driver’s License, Voters ID, PAN card, Aadhar card or a copy of a bank passbook. As a proof of address, documents like your recent landline or mobile bill, electricity bill, passport copy, recent Demat account statement, latest bank passbook, ration card, Voter ID, rental agreement, Driving License, or Aadhaar card.
The process can currently be done via the following channels -
- Offline: This involves the customer downloading the KYC application, filling in the details in a physical document, signing the document and submitting it to the specified authorities along with the attested copies of the required documents.
- Online: By uploading said documents and filling in the details required in an online form. These documents and details are verified by manual reviewers who will, depending on the image quality of uploaded documents and if the details on the documents are verifiable, correspond with the customer to finish the process.
The KYC/KYB process for a business involves a different set of documents and information for verification and risk assessment like
- Company name, seat and address
- Proof of address like passport, credit card statements, etc
- Legal firm, the name of the legal representative e.g director
- Jurisdiction under which the company is incorporated
- Names of the beneficial owner (if they hold at least 25% of the shares)
- Commercial register entry
- Commercial register number and form
- Shareholder list
- Transparency register entry
- Proof of ID of the beneficial owner and the legal representative
Learn more about Enhanced CDD best practices here.
Regulatory risks - Should you automate?
These processes are inefficient, time consuming and can still lead to errors meaning the chances of fraudulent transactions going through is still high. We will talk more about the problems in the current procedures in the following section. The errors, the time taken as well as response mechanisms can be made better by using deep learning methods to automate KYC procedures. But when a company decides to automate the entire onboarding process, there are a few regulatory risks that come with it that have to be mitigated.
Financial inclusion
One such risk is financial inclusion of undocumented people or people who come from rural areas and cannot furnish the documents required. Some of the challenges in financial inclusion as mentioned in these FATF guidelines are shown below.
- Undocumented people are barred from availing services.
- Lack of familiarity and knowledge about financial services.
- People who have mismanaged their finances in the past or have irregular income cycles may get classified as high risk individuals according to the directives.
- AI trained on unbalanced data can lead to a racial or gender bias in the detection process, leading to people otherwise eligible to avail financial services might get barred from doing so.
- Cultural mistrust of mainstream financial institutions. These individuals may come from countries where banks are not safe places to deposit funds.
- Low income populations and low density areas are not attractive for the financial service provider and hence leads to exclusion.
- Regulatory frameworks often vary with jurisdictions and are not always directly adaptable to local contexts.
Data privacy
Another risk is that of privacy. Complying with AML guidelines while also respecting customer privacy can seem at odds with each other. While AML guidelines requires a company to extensively understand their customers by using their personal information, draw insights on their behavior and predict the risks associated with doing transactions with them, privacy directives like GDPR significantly restricts how the data is acquired, used and managed. If KYC data is compromised due to improper adherence to security policies, the penalties under GDPR can be very stiff — up to 2% of global annual revenue, in some cases.
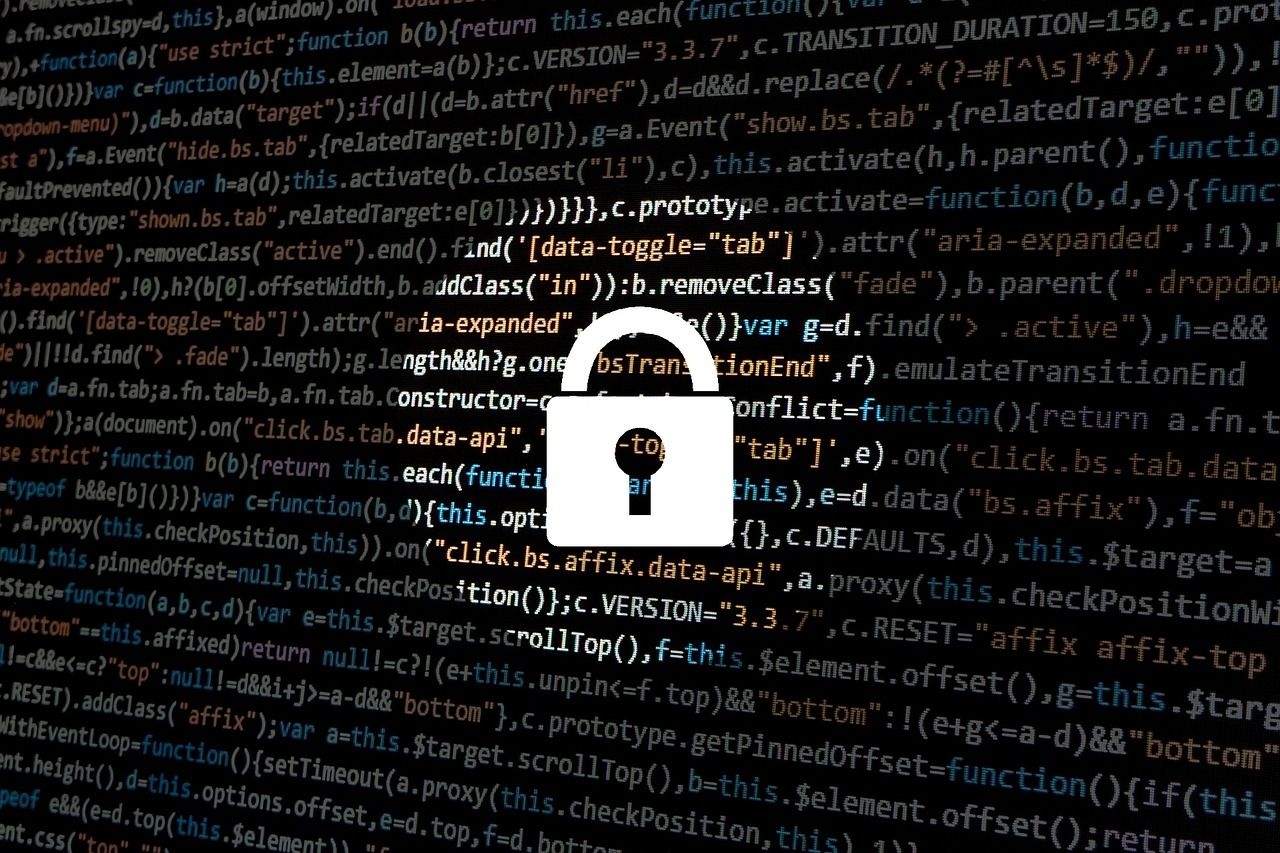
A company needs to be open and transparent about its KYC procedures, and compliance requirements and keep its customers fully informed about their involvement and how their data is being used. A few guidelines to make this work are
- Document the legal basis for processing personal data for KYC purposes
- Send privacy notices to customers and beneficial owners
- Keep customer files accurate and up to date
- Secure personal data
- Give customers more control over their information after onboarding
To learn more about incorporating data privacy in your AML and KYC compliance programs check out this white paper, or this article or this article.
Country risks
The Habib Bank case serves as a good example of how bad regulatory practices with regard to transactions in different countries can lead to heavy fines ($225 million in this case) and losses for the bank. HBL excluded certain high risk countries where it had its group offices from the list of high risk countries it maintained, claiming it had local knowledge of the areas and the countries due to the group office establishments, a claim the regulators found to be misconceived.
Financial institutions should make sure to assess risks of doing transactions in different countries and make sure to follow directives like the FATF guidelines in assessing high risk countries and areas depending on illicit drug trade susceptibility, strong links to terrorism financing, areas involved in the proliferation of weapons, etc.
Where the current workflows lack
An increasing number of businesses that need to be AML and KYC compliant are adopting a semi-automated approach to gathering and evaluating documents.
Take for example a bank providing accounts and mutual funds services or an insurance company.
At the time of opening of savings bank account, fixed deposit, mutual fund, insurance, etc, the customer needs to submit address proof and a photograph. These documents are PAN Card, Driver’s Licenses, Aadhar Card, etc. Insurance companies have to verify each document by checking the details and finding out if the documents are fraudulent.
Time-consuming
This is a time-consuming process and requires manual reviewers. The customer has to upload documents, fill in required information and wait for the completion of the review process before he/she is successfully onboarded. This process can take anywhere between a few days to a few weeks.
Possibility of fraud
Detecting fraud customers requires the manual reviewers to understand the ways an image can be manipulated, needs to verify the information extracted against various databases to make sure it is legitimate and to some extent might have to guess if the photograph or a signature is fake. The manual reviewers can themselves not guarantee 100% accuracy on whether a photograph or a signature is fake or in their data entry processes.
Image quality check
When a potential customer uploads documents as images, whether the verification, information extraction, data entry and fraud detection are done correctly depends heavily on whether the image quality is good, the image is sufficiently high resolution, in the right orientation and follows the right template. In absence of these, the verification process becomes vulnerable to errors, which can mean losses for your company and can pose a reputational risk.
Manual verification
Manual verification is a part of most KYC processing workflows and they aren’t the most efficient. Several reviewers have to go through several documents, make sure the information is correct and check for fraud. Humans aren’t anywhere close to being as fast as computers and automating this process can mean a lot of time and money saved for the company, a higher rate of onboarding, and better employee satisfaction.
Manual data entry
All the information that in submitted to an organization needs to be entered into some software that makes storage and indexing of this information possible. This data entry process is handled by humans who type in what they read in a document. This is indeed a very slow process, requires several people working for several hours and is error prone. The errors can be due to bads images, wrong data provided or employee fatigue.
Hurts onboarding volumes
Having to go through a long process of uploading documents, having them verified, making corrections in the entries made by the organisation before a customer gets onboarded, a process that typically takes more than a week hurts the amount of people who go through with the entire process.
Hurts customer satisfaction
A process that takes long, can still lead to errors and can lead to legitimate customers seem fraudulent and get off-boarded anytime leads to unsatisfied customers. This can change by using smarter algorithms that can see the onboarding process to the finish with high accuracy and fast speeds.
Solving the KYC automation problem
To automate the process of customer onboarding for different organisations involves several steps and requires us to be careful about our model metrics and performance so we can make sure the organisation can cut costs while increasing efficiency in the process.
Automate image quality checks
Several times, a bad image can delay the verification process by days or weeks. The user has to upload new images after the company informs them about the bad image quality. There are several factors to consider while checking image quality as well which are better done with computer vision algorithms than manual reviewers. This also allows us to provide customers with immediate feedback about the images, whether they are blurred, in the wrong orientation, etc. Getting immediate feedback means customers can now finish up the document uploading in minutes instead of waiting for days to get a confirmation from the company.
Automate document verification
Once good quality images reach the company, these images have to be verified against the right document templates and manual reviewers need to confirm that all the information required is present in the uploaded files. This again might cause a delay in the process if the documents are wrong, not attested, do not have all the necessary information, etc. Using object detection and OCR models that are trained on a broad set of forms and documents data can help verify if, for example, the image shown is of a driver’s license or a passport, if all the required fields are entered in the document.
Automate fraud detection
Ensuring compliance means making sure that defaulters are kept away from making legitimate transactions using false information which requires companies to weed out the people who act suspiciously. This process when done by humans is not very reliable since digitally manipulated images can easily fool a human and can cause fraudulent transactions to go through that might cause fines or reputational damage to the company for the lack of strong compliance mechanisms and negligence. To avoid this, training and using machine learning models to detect fraud in a human moderated loop can significantly increase efficiency and reduce the possibility of fraud.
Automate document digitization
Having checked image quality, verified the documents and checked for fraud, these documents usually go through the hands of a person who will read these documents and enter the information needed into a software so it is indexed and stored into a database. This process can again be automated. With the right object detection and OCR models, fields can be identified, localized and the text in them can automatically be extracted and entered into databases with little or no human intervention with close to 90% reduction in the time consumed.
The Nanonets API
And this is where the Nanonets API shines. With Nanonets you do not have to worry about finding machine learning talent, building models, understanding cloud infrastructure or deployment. All you need is a business problem that you need solutions for.
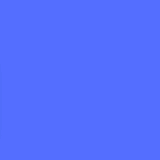
Easy to use web-based GUI
Nanonets offers an easy-to-use web-based GUI that communicates with their API and lets you create models, train them on your data, get important metrics like precision and accuracy and run inference on your images, all without writing any code.
Cloud-hosted models
Besides providing several models that can be used out of the box directly to get solutions, users can build their models that are hosted on the cloud and can be accessed with an API request for inference purposes. No need to worry about getting a GCP instance or GPUs for training.
State-of-the-art algorithms
The models built using state-of-the-art algorithms to get you the best results. These models constantly evolve to become better with more data and better technology, better architecture design, and more robust hyperparameter settings.
Intelligent field extraction
The greatest challenge in building an invoice digitization product is to give structure to the extracted text. This is made easier by our OCR API which automatically extracts all the necessary fields with the values and puts them in a table or a JSON format for you to access and build upon easily.
Automation driven
We at Nanonets believe that automating processes like invoice digitization can create a massive impact on your organization in terms of monetary benefits, customer satisfaction, and employee satisfaction. Nanonets strives to make machine learning ubiquitous and to that end, our goal remains to make any business problem you have solved in a way that requires minimal human supervision and budgets in the future.
OCR with Nanonets
The Nanonets OCR API allows you to build OCR models with ease. You can upload your data, annotate it, set the model to train and wait for getting predictions through a browser-based UI without writing a single line of code, worrying about GPUs or finding the right architectures for your deep learning models.
Using the GUI: https://app.nanonets.com/
You can also use the Nanonets-OCR API by following the steps below:
Using Nanonets API
Below, we will give you a step-by-step guide to training your own model using the Nanonets API, in 9 simple steps.
Step 1: Clone the Repo
git clone https://github.com/NanoNets/nanonets-ocr-sample-python
cd nanonets-ocr-sample-python
sudo pip install requests
sudo pip install tqdm
Step 2: Get your free API Key
Get your free API Key from https://app.nanonets.com/#/keys
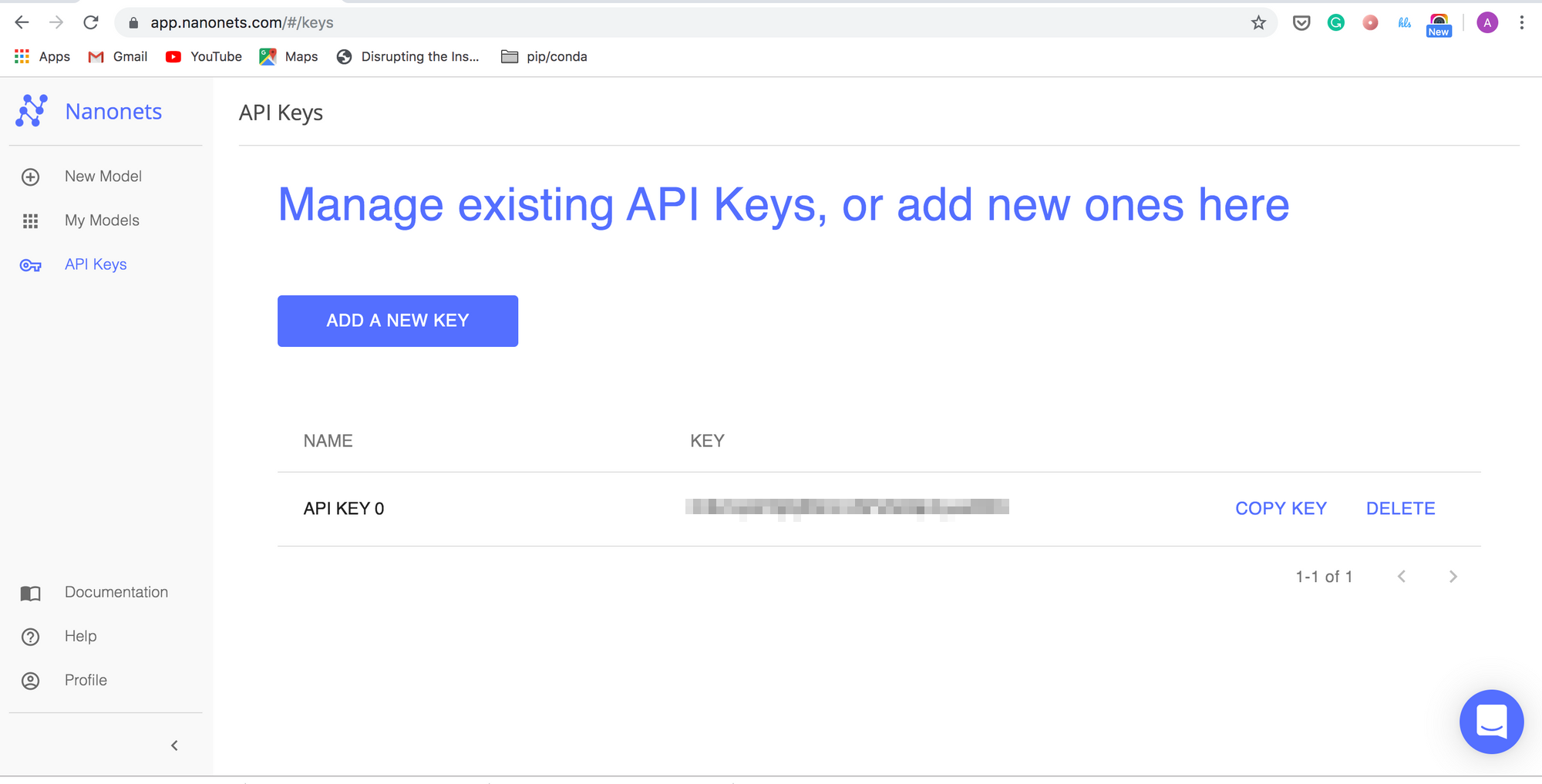
Step 3: Set the API key as an Environment Variable
export NANONETS_API_KEY=YOUR_API_KEY_GOES_HERE
Step 4: Create a New Model
python ./code/create-model.py
Note: This generates a MODEL_ID that you need for the next step
Step 5: Add Model Id as Environment Variable
export NANONETS_MODEL_ID=YOUR_MODEL_ID
Step 6: Upload the Training Data
Collect the images of object you want to detect. Once you have dataset ready in folder images
(image files), start uploading the dataset.
python ./code/upload-training.py
Step 7: Train Model
Once the Images have been uploaded, begin training the Model
python ./code/train-model.py
Step 8: Get Model State
The model takes ~30 minutes to train. You will get an email once the model is trained. In the meanwhile you check the state of the model
watch -n 100 python ./code/model-state.py
Step 9: Make Prediction
Once the model is trained. You can make predictions using the model
python ./code/prediction.py PATH_TO_YOUR_IMAGE.jpg