Business Process Outsourcing (BPO) Automation
What is a BPO?
In general, the idea of outsourcing is to use outside vendors to carry out standard business functions that are not core to the business . This simplifies the management task, allowing for the company to retain only core staff with focus on the high-value activities of growing the business and researching new opportunities, while regular and well-understood operations, like manufacturing and workflow management, can be delegated to external vendors.
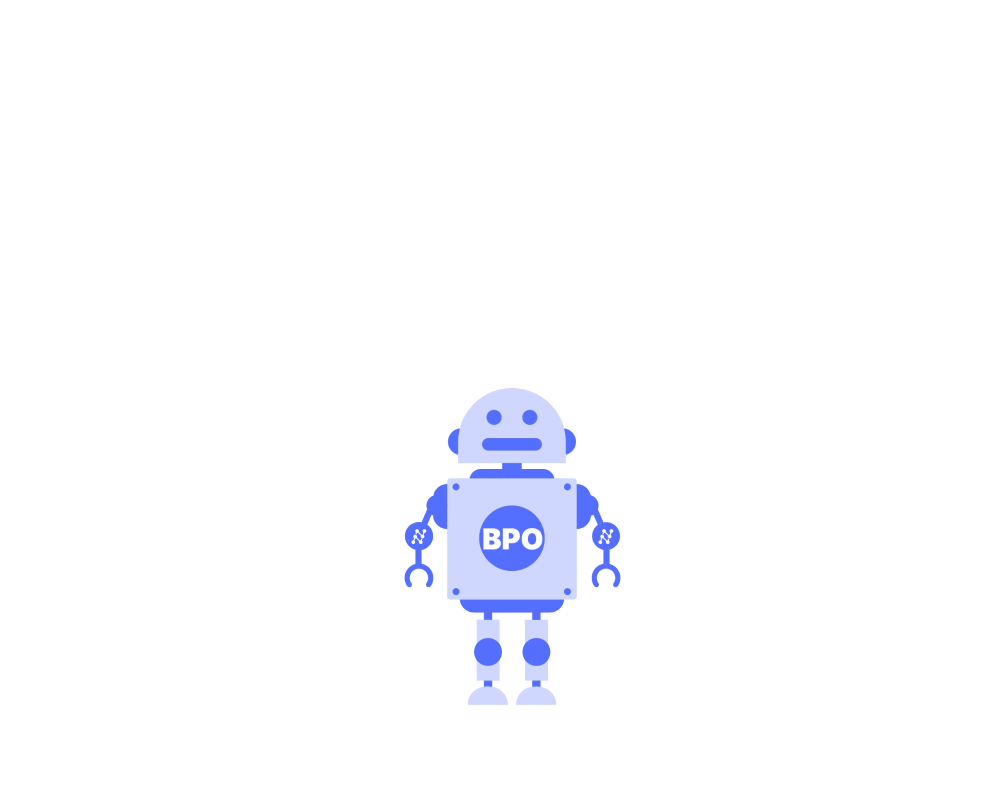
Overseas vendors are often favored, because they bring competitive advantages to the combined enterprise, like lower labor costs for vertically-specialized workers, multilingual skills, overnight operations or better disaster-recovery response due to geographically distributed operations.
The industries that need to process a large daily volume of paperwork spend much effort and money managing workflows. A workflow consists of a sequence of administrative checkpoints and actions, each performed by a different worker, like the steps involved in paying an invoice or approving a health insurance claim.
- What is a BPO?
- Automation in Business Process Outsourcing (BPOs)
- BPO Fulfillment Services
- Impact of Artificial Intelligence on BPO Services
- A Brief History of AI
- You Have the Right to an Explanation
- Examples of BPO Offerings Incorporating AI
- Other AI Opportunities in the BPO Industry
- Conclusion
Automation in Business Process Outsourcing (BPOs)
The manual and repetitive nature of the tasks embedded in a workflow often lead to human errors and data loss causing delays and re-work. This is magnified in complex businesses operated at large scale. All the above makes workflow management a good target for automation via software. Computers will perform the repetitive tasks without introducing random errors due to human attention fatigue.
An example of a repetitive task is Data Entry. In the case of the health insurance claim process, the workflow begins with a staff member uploading scanned images of paper documents to a cloud storage. The next step involves a worker who looks at the document image, reads it, interprets it well enough to understand the relevant pieces of information, and types them into a system for storage as numeric and text fields and further processing by the subsequent steps of the workflow.
The Data Entry task can be automated with the help of Optical Character Recognition (OCR) and Information Extraction (IE) technologies (see [1] for an in-depth technical example), eliminating the risk of human errors.
Looking for an AI based solution to enable automation in BPOs ? Give Nanonets™ a spin and put all document related activities in Business Process Outsourcing on autopilot!
BPO Fulfilment Services
Since the early 1990s, supply chains have been run to maximize their efficiency, driving the concentration of specialized services in providers that offer economies of scale. For example, the iPhone supply chain comprises vendors in up to 50 countries. This globalization of the manufacturing networks has a parallel in the business processing world, as companies have learned to rely on BPO fulfillment vendors from across the globe.
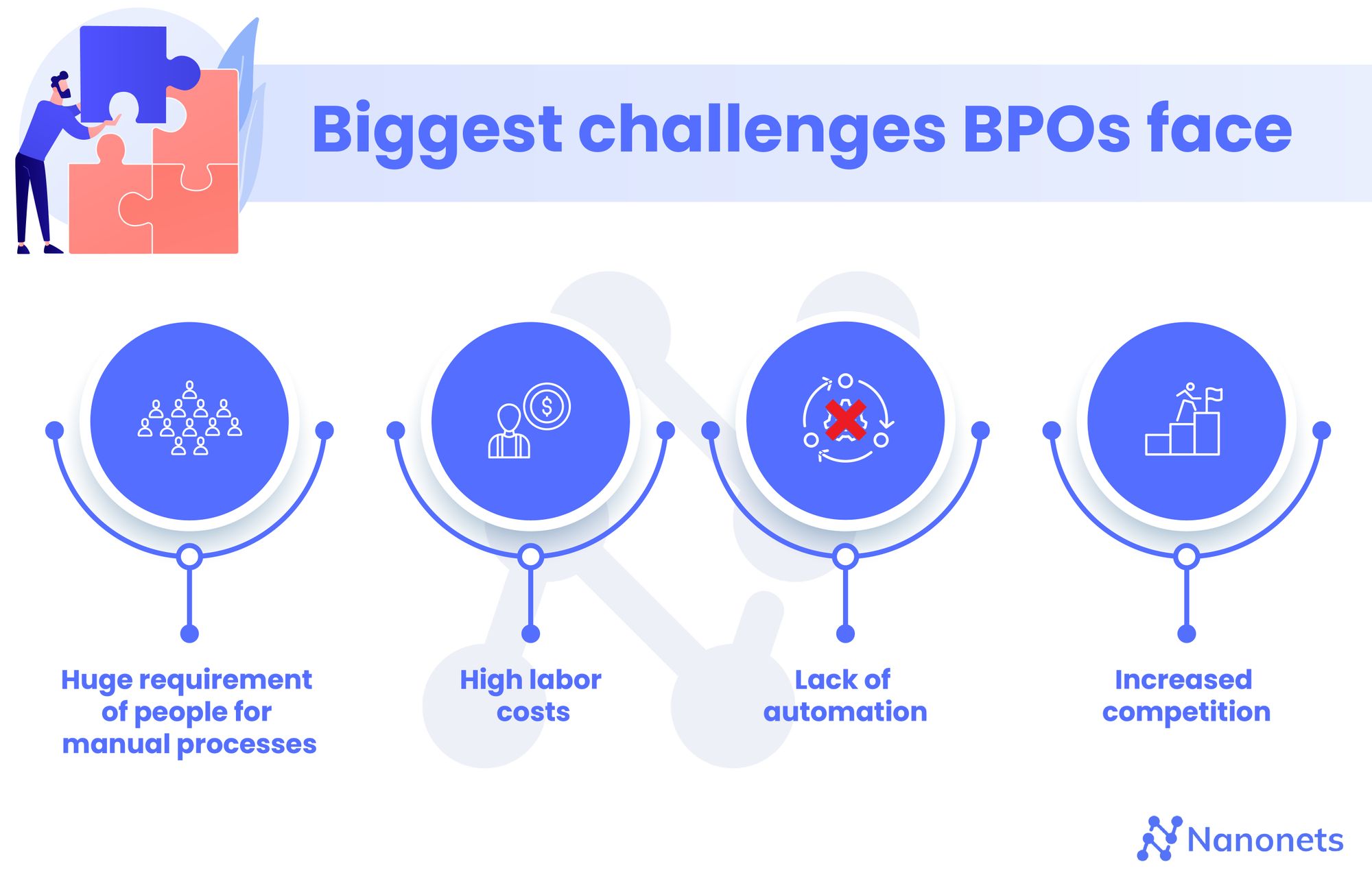
BPO not only is indispensable to a small company that wants to capitalize on a sudden surge in demand of its products, but it also makes sense for most companies. For example, consider outsourcing activities like telemarketing, that is why many BPO companies offer services on lead generation, sales, and customer service. Although BPO Fulfillment has already become a multi billion dollar industry, its growth may accelerate with the adoption of AI technologies.
Impact of Artificial Intelligence on BPO Services
Onshore AI-powered solutions now present viable alternatives to the traditional offshore BPO services with:
- Equivalent levels of quality and superior geographic independence
- Labor cost efficiency
- Processing speed
- Scalability
- Accuracy
- Carbon footprint
Considering that only 10 years ago AI was not even in this race, many observers can foresee that in the near future most BPO will be making the transition to partial or fully AI-powered offerings.
(source: https://enterprisersproject.com/article/2020/1/rpa-robotic-process-automation-5-lessons-before-start)
A Brief History of AI
For the last 20 years, AI-powered retail and marketing has enjoyed great success. The mining of actionable insights from customer behaviour data captured all over the internet has done the trick for most companies allowing them to maximize the ROI of their retail operations and marketing investments.
The data that defined AI in the decade of the 2000's was tabular, this means data neatly organized in columns and rows. That explains why the first wave of commercial AI was limited to processing spreadsheet-like data (just bigger), it was the golden era of:
- recommender systems based on collaborative filtering algorithms
- search portals powered by graph algorithms
- sentiment and spam classifiers built on n-grams
In the next decade, the 2010s, commercial-grade AI broke the tabular data barrier, beginning to process data in the form of sound waves, images and to understand simple nuances in text or conversation.
This was enabled by the development of deep neural networks, a new breed of bold and sophisticated machine learning algorithms that power most of today's AI applications and that, given enough data and computing resources, do everything better than the previous generation, plus hear, see, talk, translate and even imagine things.
All this progress was based on machine learning systems that codify their knowledge into millions (sometimes billions) of numeric parameters, and later make their decisions combining those parameters though millions of algebraic operations, making it extremely hard or practically impossible for a human to track and understand how a particular decision was made, this is why those models have been characterized as black boxes, and the need to understand them has motivated the study of a new buzzword: Explainable AI or XAI for short.
(source: https://www.kdnuggets.com/2019/12/googles-new-explainable-ai-service.html)
Looking for an AI based solution to enable automated business processing ? Give Nanonets™ a spin and put all document related activities in Business Process Outsourcing on autopilot!
You Have the Right to an Explanation
With AI enjoying more attention from academia and investors like never before, it will continue to improve its human-like abilities, and now it is time for it to grow enough sense of responsibility and civic duty before it is put in charge of deciding who gets a loan or advicing on which patients can be discharged from a hospital.
The main concern is that decisions about human subjects become hidden inside complex AI-powered decisions that no-one cares to understand, as long as the decision appears to be optimal, even if it is based on racial bias or other socially damaging criteria.
In this regard, one of the most active areas of research in AI is about developing tools that allow for humans to interpret model decisions with the same clarity that all human-made decisions within an organization can be analyzed.
This is known as right to explanation and it has a sensible and straightforward expression in countries like France who have updated a code from the 1970s aimed to ensure transparency in decisions made by government functionaries, by simply extending it to AI-made decisions. These should include the following:
- the degree and the mode of contribution of the algorithmic processing to the decision- making;
- the data processed and its source;
- the treatment parameters, and where appropriate, their weighting, applied to the situation of the person concerned;
- the operations carried out by the treatment.
(source: https://www.darpa.mil/program/explainable-artificial-intelligence)
A more practical concern raised by AI-based BPO is about liability, who is ultimately responsible for a failure of the AI system?
Finally, there is the question of intellectual property, as AI systems can learn from experience, who is the owner of the improved knowledge that the AI system has distilled from the data produced by the BPO customer operation?
These concerns have clear implications for AI-powered BPO, which may need to address them in service contracts.
Looking for an AI based solution to enable automation in BPOs ? Give Nanonets™ a spin and put all document related activities in Business Process Outsourcing on autopilot!
Examples of BPO Offerings Incorporating AI
Although AI is currently unable to match humans in mental flexibility to deal with new situations or even to have a child-level understanding of the world we live in, it has demonstrated ability to perform well in narrowly-defined knowledge domains, like the ones that make good candidates for BPOs.
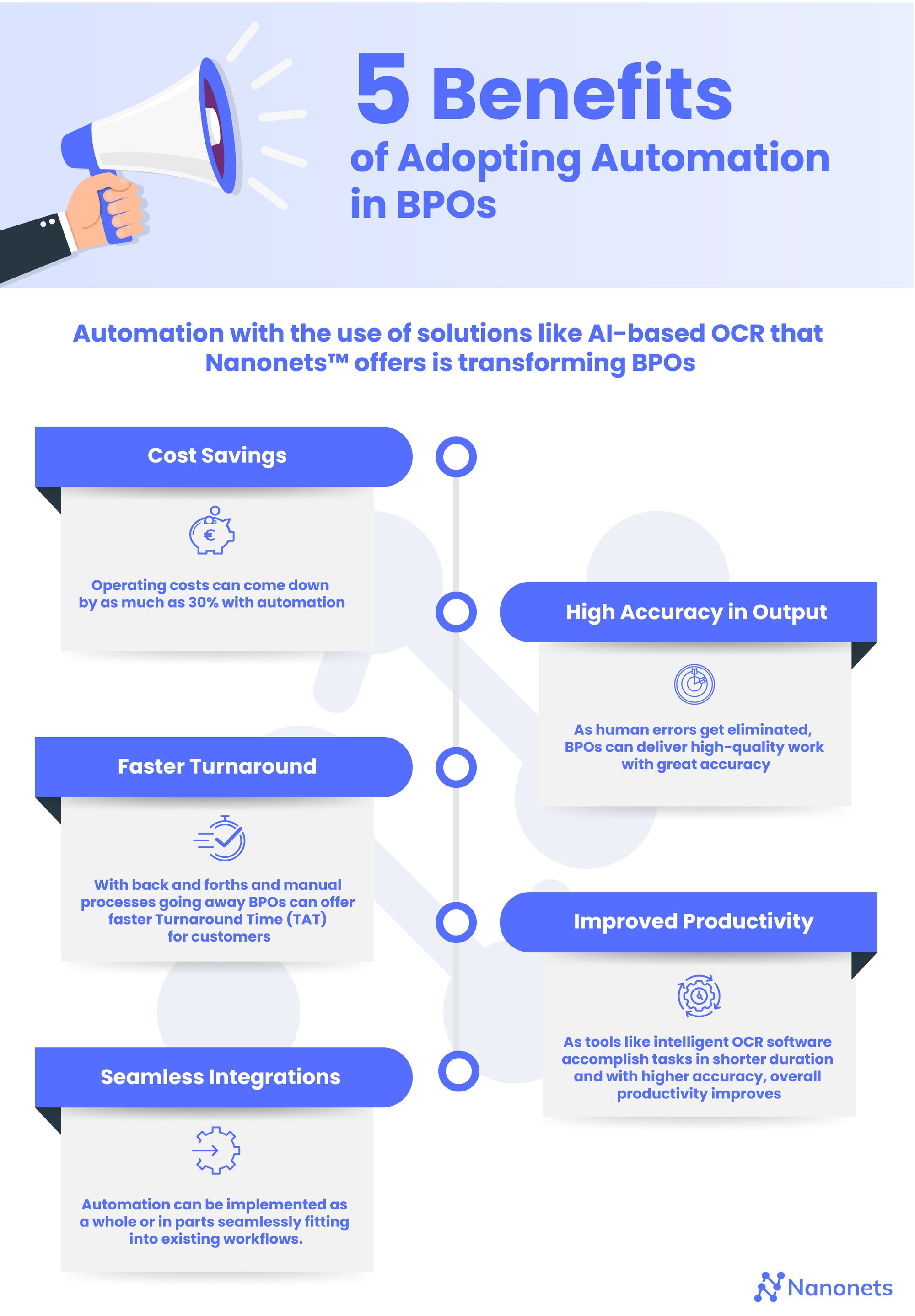
Document Management
A large vertical of the BPO Industry is Document Management, this sector is undergoing a massive transformation as large companies in document-centric industries standardize and document their internal processes getting ready to outsource them in order to focus on their core competencies.
This trend is driving traditional document management service providers to develop a more sophisticated service offering, including business verticals like:
- Invoice Processing
- Digitization of Healthcare Records
- Claims Processing
- Bank Statement Ingestion
- Loan Application Processing, etc,.
As these BPO providers evolve beyond their basic services of document scanning and archiving, with the occasional reporting and printing, towards higher value-added services, they need to develop an integrated stack of technologies, comprising high-speed and high-volume document scanners, advanced document capture, data recognition and workflow management software.
The AI-powered data extraction from scanned images is the key that opens the door to high-value added services like ARP. In the image below we see how an AI system sees an image. To the AI model, the image is represented by a group of text areas and the relative distances between these areas. With this information the system applies statistical inference to arrive to the most likely meaning of each text and number in the image.
(source: https://nanonets.com/blog/information-extraction-graph-convolutional-networks/)
Consumption of ML service through API
The above discussion explains the need and value of integrating AI services with the document processing workflows. In this section, I discuss the integration of a cloud-based OCR and Data Recognition service. This service extracts all the interesting information from a scanned form, and returns it in computer-readable format to be further processed by workflow management software.
This AI component comprises two workflows: the first one trains a machine learning model with examples provided by the model developer, and the second workflow just calls the model trained in the first step to extract information from the documents.
In consideration of the readers' time constraints, I only show an outline of the main steps involved in a typical process.
Training Workflow
- Step 1: Document Scanning. This process converts a physical paper document into an image file, in a standard format like JPEG, PDF, etc.
- Step 2: OCR processing. This process recognizes areas of the document containing letters and digits and outputs their contents as a list of text segments, together with their bounding box coordinates.
- Step 3: Manual annotation of the images: this process is performed manually by a human with the help of a special editor that allows to select an area of text and assign a tag which basically identifies the type of information contained in the text, for example the date of purchase in an invoice, etc.
- Step 4: Upload the examples to the could. This process is performed by calling an API and has the purpose of making the training examples available to the AI cloud software so they can be utilized in the next step to train the model.
- Step 5: Train an Information Extraction Model. This process is triggered by calling an API. After the training is completed the model is available to be used in the production workflow.
Production Workflow
This is the workflow that produces useful results on the customer data. The first two steps are common with the training workflow, the difference starts in the 3rd step, which in the language of machine learning is known as "prediction" (although its meaning is closer to "making educated guesses").
- Step 3 (predict): Automatic Information Extraction: this process is performed in the cloud by the AI model. The model goes over the OCR output and recognizes the numbers and text segments that are useful for further processing. The output can be tabular data, in a format that is easy to process by the software that performs the next task in the workflow.
Looking for an AI based solution to enable automation in BPOs ? Give Nanonets™ a spin and put all document related activities in Business Process Outsourcing on autopilot!
Other AI Opportunities in the BPO Industry
The opportunities to apply AI in the BPO industry usually fall in two large categories: robotic process automation (RPA) and chatbots.
What is RPA?
In the context of BPOs, robotic means the kind of technology that automatically makes decisions about finance and accounting spreadsheets. In this category, we can include a huge number of insight-mining services that are commonplace in most large-size companies but not yet affordable to all, like customer personalization based on recommender systems, classifiers that approve loans or detecting churn in customer accounts, also text classifiers to categorize customer feedback into positive or negative, or even classifiers to detect bots and trolling in the company's social media. This is in parallel to the processing of data from sensors and the subsequent computation of analytic indicators that drive efficiencies in the supply chain.
(source: https://www.processmaker.com/blog/how-do-banks-benefit-from-robotic-process-automation-rpa/)
Conversational Agents
Generally known as "chatbots", conversational agents can be divided in two large categories: task-oriented and chatbots.
A chatbot just makes up conversation and it can be found in social media participating in blogs. It is expected to express opinions on a wide range of topics of which it knows nothing about, a famous case of Microsoft’s Tay bot is a typical example of this category (although this one did not have a happy ending, it was a great lesson on the subtleties of AI adoption).
On the other side of the spectrum, we find task-oriented agents, whose only mission is to handle some practical task in a specific domain, this would be the case of your phone handling a restaurant booking or routing you to a destination.
With enough training, task-oriented agents can diligently handle the most common questions processed in a telephone help desk and customer contact services. The chatbot can only operate satisfactorily in a narrow field of knowledge and for that reason is usually deployed as the first tier that tries to answer simple cases and for all other issues it tries to route the thread to a human specialist.
Even though the chatbot may not be able to answer a wide variety of questions, (questions that fall in the "long tale" of the distribution) just by answering the most frequent ones it has a valuable impact on reducing the number of calls answered by humans.
Conversational agents have come a long way but they are still an area of research, there is progress in certain areas, with impressive AI designs published in scientific papers but very limited application in industry due to the difficulty in acquiring high quality datasets. The typical workflow of a conversational agent can be seen below.
(source: https://arxiv.org/abs/1703.01008)
The Data Trove
We have developed AI systems that are able to absorb knowledge specific to a field of business, and we have also developed sophisticated ways to represent that knowledge in a way that machines can process it.
Now, all we need is a dataset, a properly annotated and depurated set of data, with lots of examples and enough details for the AI system to learn. This proves to be one of the most important challenges, given that AI systems do not learn like humans from just a few examples.
AI typically needs many examples of every little thing you want it to learn. If your data presents a large variety of cases, then you need several examples of each one of these cases. And then, human language has tens of thousands of cases called words, which can be combined to form a huge number of sentences and express a innumerable number of concepts. Sentences in turn can be combined to form conversations.
At this point you see why acquiring conversational datasets can be a challenge. Luckily for us, we don't need to train an AI system from scratch. Thanks to a technique known as transfer learning, we can start from a system that already understands language, and all we need to teach is the meaning of words in a vertical business.
The cost of conversational dataset development makes this type of AI systems prohibitive for small companies to train and they remain only affordable to the largest data powerhouses. This is why some of the most operationally-significant break-throughs in conversational agents research consist not so much in model development but in the design of a training mechanism that makes efficient use of the dataset.
(source: https://arxiv.org/abs/1703.01008)
This involves the use of simulators, that are able to generate new combinations of sentences in the dataset and effectively multiplying the size of the dataset. The diagram above depicts a workflow used to train a goal-oriented conversational agent with the help of a rule-based simulator that combines the sentences from a static dataset according to some simple hard-coded rules.
The chart below shows the performance of an AI system learning to converse with a rule-based simulator. After a number of training episodes, the conversational agent catches up and surpasses its rule-based trainer.
(source: Spoken Dialog System trained with user simulator)
Beyond Chatbots
Omnichannel call centers are a recent evolution of the traditional call center, these are services that manage customer communications across multiple channels, including emails, documents, voice calls and chat sessions.
A huge opportunity lies in harnessing the data collected along with the business processes (or business rules) to maximize process efficiency. For example, by extracting insights from customer communications, companies can personalize marketing and customer services, which has the potential to dramatically maximize the marketing ROI.
But in order to extract actionable insights from multichannel customer threads, it is not enough to store the media in a central repository, an AI stack is needed to extract readable text and also to properly contextualize the communications for example, detecting sentiment or emotional content.
This has motivated the integration of the call center software with AI technologies like natural language processing (NLP) and voice analytics that examines vocal tone in audio or emotional clues in video.
Although most management leaders are only starting to figure out how to gain access to it, AI has the power to transform the heaps of disparate media generated by omnichannel call centers into a valuable trove of interpretable and actionable insights that enable the company leadership to apply data-driven management techniques.
For example, the company can undertake root-cause analysis of agent performance by engaging NLP algorithms to find out if they are empathetic to customers, or whether they follow call scripts and observe company policies. These are actionable insights that can guide decisions about training, hiring and performance management of agents. The same data can be analyzed to make product and process improvement decisions to minimize support calls.
Looking for an AI based solution to enable automation in BPOs ? Give Nanonets™ a spin and put all document related activities in Business Process Outsourcing on autopilot!
Conclusion
We have discussed AI integration in the BPO software stacks of different BPO sectors, including omnichannel call centers, document processing and workflow management.
The integration of AI is going to prove essential for the BPO services to continue developing high value-added services to satisfy the evolving demand of their customers.
In response to this huge potential market, some AI companies are specialized in document processing and NLP functionality over SaaS platforms that are easy to consume through a simple cloud API.
Nanonets has perfected an OCR + IE stack and packed it conveniently behind a high-performance service API, for the developers of workflow management software to take advantage of it without having to incur the costs of building and maintaining this highly specialized stack of AI technologies.
Nanonets is committed to continue developing an AI platform, with the added benefit of an active online community of users and a strong network of partners offering solutions, consulting, and training.
Update: Added more info based on queries from users.