Data Annotation – Definition, Tools & how to automate it?
Looking to automate data annotation?
Try Nanonets for free. Create custom workflows to automate manual data processes in 15 minutes. No credit card is required.
Machine Learning and Artificial Intelligence are rapidly growing technologies giving rise to unbelievable inventions delivering advantages to several fields globally. And to develop such automated machines or applications, an enormous amount of training data sets is expected.
That’s where data annotation comes into play. Data annotation helps make sense of data and is used extensively by businesses for multiple use cases.
Let’s see how it works and how you can automate it.
What is data annotation?
Data annotation is the process of labeling information within videos, images, or text. This labeling facilitates model comprehension of a data source, enabling recognition of specific formats, objects, information, or patterns in subsequent analyses.
And to equip computer vision with an established machine learning model, it needs to be precisely annotated using adequate tools and methods. And numerous types of data annotation methods are used to develop such data sets for such necessities.
What are the types of data annotation?
Data can be of multiple types: audio, text, image, and video. For every type of data, we have to perform data annotation. So, let’s see different types of data annotation.
Text Annotation
70% of companies are dependent on text.
The most generally used data category, and if you need to use text efficiently, your AI models should understand what text is written. That’s where text annotation comes into play.
Text annotation labels and provides metadata for your textual data. This means you’ll label the text and tell the AI what the text is saying. It can add information about the text's meaning, structure, or sentiment, among other things.
We will discuss the following types of text annotation:
- Sentiment Annotation,
- Intent Annotation,
- Semantic Annotation,
- Named Entity Annotation,
- Relation Annotation
Sentiment Annotation
Sentiment annotation helps to identify and categorize the human emotions, attitudes, and opinions expressed in any kind of text. Human annotators are often used to assess sentiment and appropriate content on various web platforms, including social media and e-commerce sites. Sentiment annotation identifies and flags sensitive or offensive content and words.
For example, if you were to annotate the sentence, " I enjoyed the birthday dinner,", you would classify “enjoyed” with a positive sentiment.
Intent Annotation
Intent annotation is necessary for human-machine interactions. It helps devices understand user intent and natural language.
Multi-intent data categorization and collection can distinguish intent into key classifications such as commands, requests, bookings, confirmations, and recommendations. It is primarily used in human-facing applications like chat support to identify what the person wants to achieve.
Semantic Annotation
Semantic annotation provides a correlation between similar items. For example, when you are searching for products on Amazon, you’ll see the “customers also liked” section. This section is created by semantic annotation.
The annotator links together products of similar nature and puts them together for customers to view. By indexing the different elements within product search queries and titles, semantic annotation services help train algorithms to understand those elements and improve overall search results.
Named Entity Annotation
Named entity annotation is a process used to identify and classify entities such as people, places, and organizations in text. NER (Named Entity Recognition) systems require a large amount of manually annotated training data.
This involves identifying and labeling entities such as people, organizations, and locations in the text.
Relation Annotation
Relation annotation involves identifying and labeling the relationships between entities in the text. For example, if you have a text like "Barack Obama is the father of Sasha Obama," you can label the relationship between "Barack Obama" and "Sasha Obama" as "father".
Audio Annotation
Audio annotation is the process of transcribing and time-stamping speech data. Audio annotation includes the transcription of speech, pronunciation, and the identification of dialect, language, and speaker demographics.
For example, this is an excellent security application. If security gadgets can identify the sound of glass breaking, they can notify the authorities.
Image Annotation
Image annotation makes it easy to comprehend visual information fed to robotics. Image annotation is essential for improving robotic vision, computer vision, facial recognition, and security solutions.
Image annotation includes providing labels to items inside the image. They can be captions, identifiers, tags, or keywords.
Video Annotation
Video usage is snowballing, and to make it safer, video monitoring applications are used to prevent the misuse of video. Video annotation helps in improving video monitoring and security applications.
Video annotation is the process of labeling items in the video with relevant tags so that AI can understand what’s in the video.
One example of video annotation in real life is in self-driving cars. To train a self-driving car to navigate roads and avoid obstacles, large amounts of video data must be collected and annotated with information such as the location of traffic lights, stop signs, and other vehicles. The car's machine learning algorithms can then use this annotated data to learn how to recognize and respond to these objects and situations in real time.
Automate any data process easily with Nanonets' no-code workflows.
Start your free trial and automate data collection, upload, verification, annotation, approvals and more.
Manual vs Automated Data Annotation
Well, let’s compare manual and automated data annotation side by side.
Which one you should opt for?
This largely depends on the type of data you’re working with. If you’re working with sensitive data, it is better to work with human data annotators to make sure there are no errors in identifying important details.
Automated data annotation is the best bet when the stakes aren’t high and the mistakes won’t lead to catastrophic events, like linking similar products together, identifying car types, or more.
Automate your manual data processes with No code workflow automation.
How to do data annotation?
Now that we’ve seen the basics of data annotation let’s learn how to do it. The steps involved in data annotation are dependent on the type of data, the scope of the project, and the specific requirements of the project. In this section, we will see a general overview of the steps involved in data annotation.
Step 1: Data collection
Before you annotate data, you need to collect it. You need to collect all the data, including images, videos, audio recordings, or text data, in one place.
A platform like Nanonets can automate data collection with data import options.
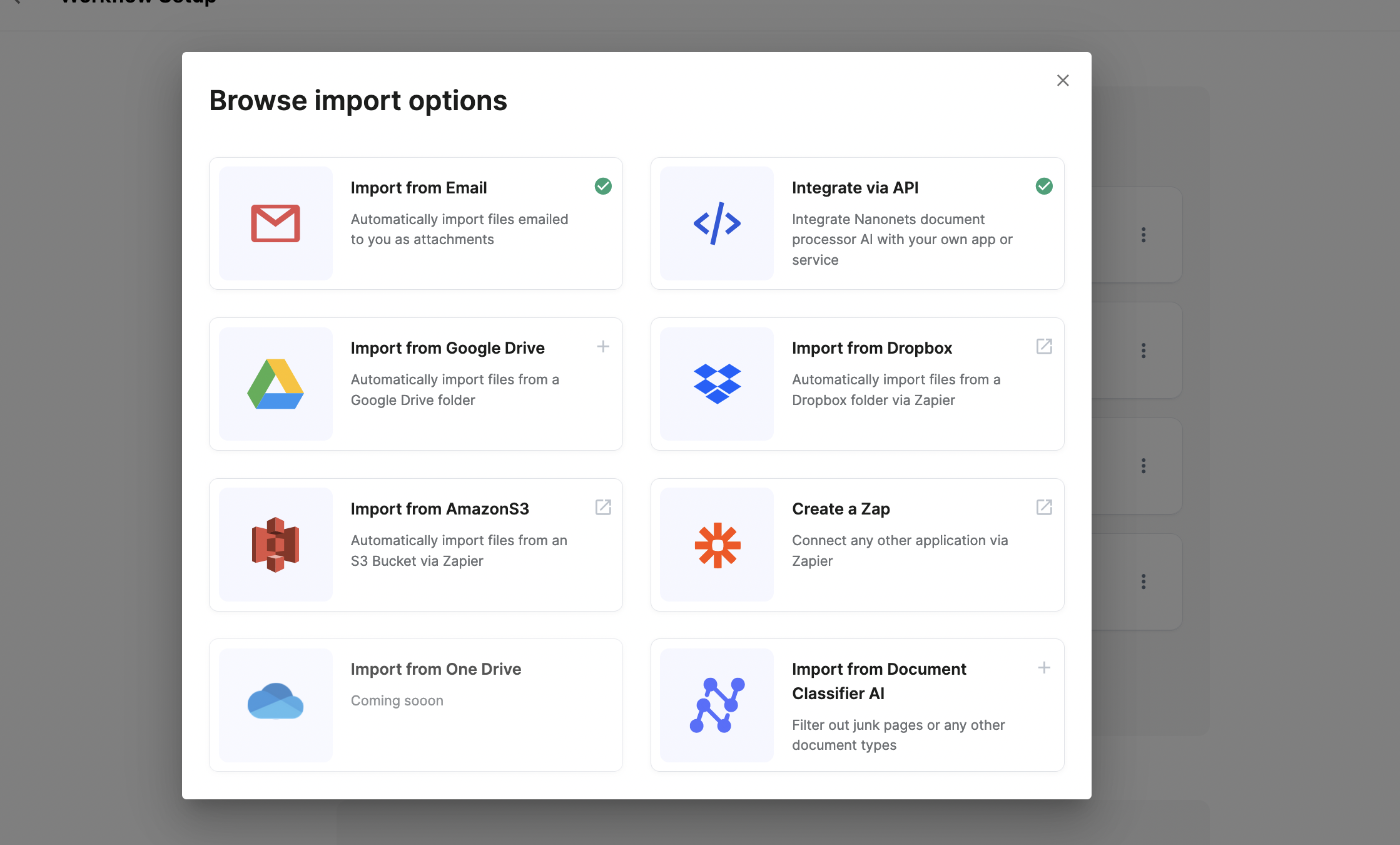
Step 2: Data preprocessing
You need to pre-process the data to standardize it. This step includes deskewing images, data enhancement, formatting the text, or transcribing video.
Nanonets can automate data pre-processing with no-code workflows. You can choose from a variety of options like date formatting, data matching, data verification, etc.
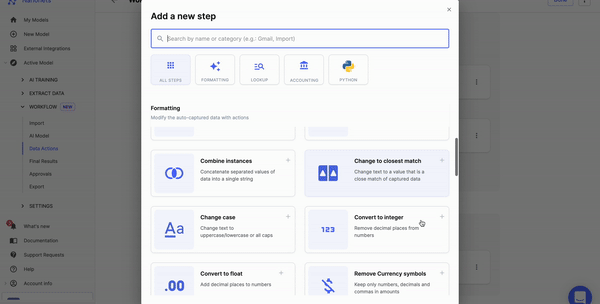
Step 3: Select the data annotation tool
Next, you should select a tool to label and tag data. Based on your requirement, you can choose the relevant tool. Here are some you can look at:
- Data Annotation - Nanonets
- Image Annotation - V7
- Video Annotation - Appen
- Document Annotation - Nanonets
Step 4: Annotation guidelines
You need to establish guidelines for annotators or annotation tools. In this case, you can make sure that there are no steps that are missed.
Step 5: Annotation
After the guidelines have been established, the data can be labeled and tagged by human annotators or using data annotation software.
Step 6: Quality control
Once data is annotated, it needs to be reviewed. You can perform multiple blind annotations to ensure that results are accurate.
Step 7: Data export
Once data annotation is done, it’s time to export it in the required format. You can use platforms like Nanonets to seamlessly export data in the format of your choice to 5000+ business software.
The entire data annotation process can take anywhere from a few days to several weeks, depending on the size and complexity of the data and the resources available.
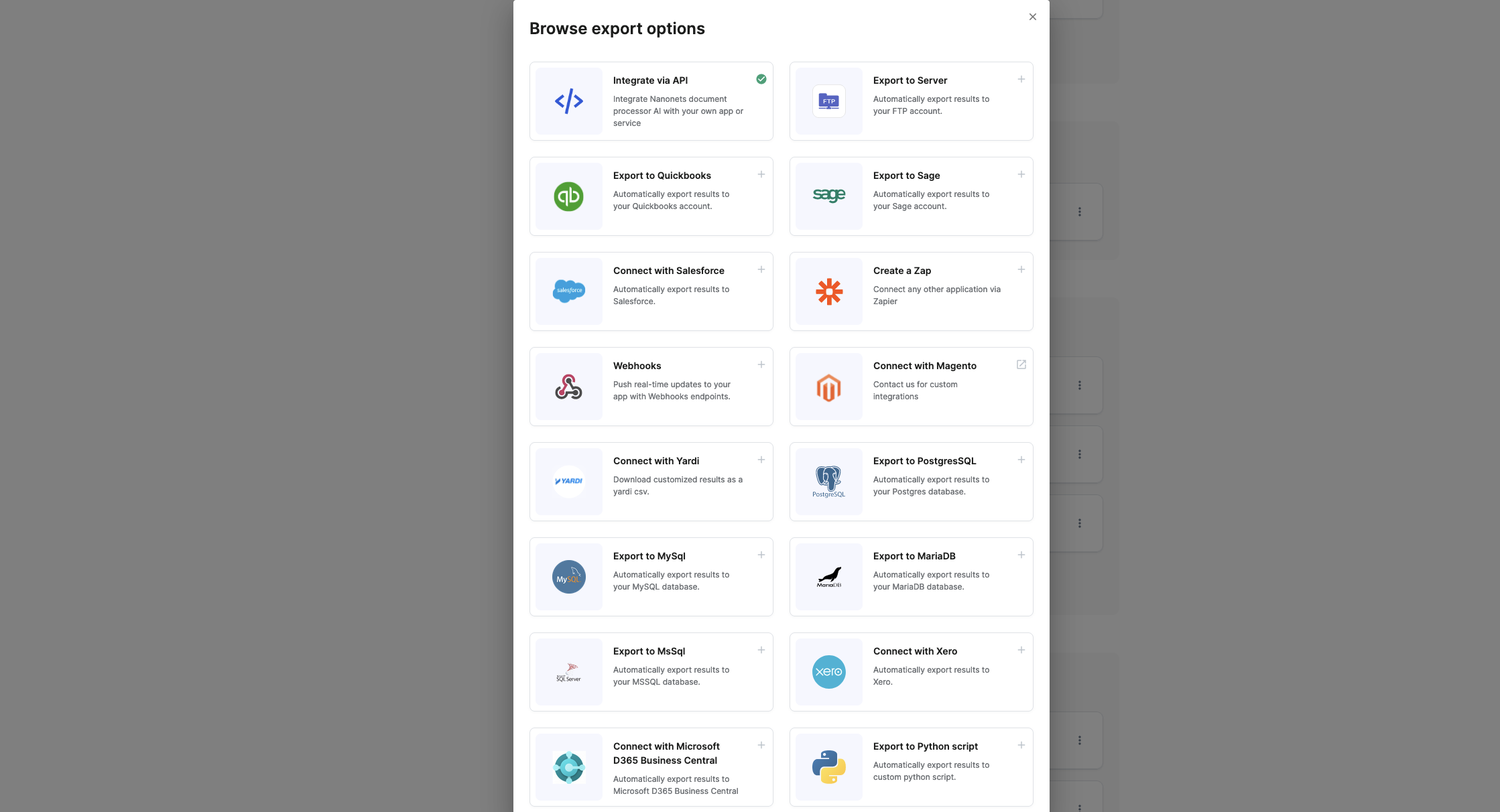
Future of Data Annotation
The amount of data generated every day is growing exponentially. It is estimated that over 2.5 quintillion bytes of data are produced every day, which is huge!
Data annotation will help businesses make sense of the data and use it more efficiently. Right now, most data annotation tools need human intervention at one stage or another. As technology advances, we might be able to automate this entire process altogether.
Software like Nanonets can simplify data annotation for businesses on the go. In case you have any document data annotation requirements, feel free to contact us. Nanonets can automate data extraction from documents and annotate documents easily to automate any document tasks.
Contact our sales team to set up no-code workflows for your use case today.
FAQ
What are different data annotation use cases?
Data annotation is beneficial in:
Enhancing the Quality of Search Engine Outcomes for Multiple Users
Search engines require users to provide detailed information. Their algorithms must filter high quantities of labeled datasets to give an adequate answer to do. For instance, Microsoft’s Bing. Back it caters to numerous markets; the vendor must ensure that the outcomes the search engine would deliver would match the user’s line of business, culture, and so on.
Improving Local Search Evaluation
While search engines seek a global audience, dealers also have to ensure that they give users localized outcomes. Data annotators can enable that by labeling images, information, and other subjects according to geolocation.
Improving Social Media Content Relevance
Just as search engines, social media outlets also need to deliver customized content suggestions to users. Data annotation can enable developers to categorize and classify content for pertinence. An instance would be classifying which content a user is inclined to consume or understand based on his or her viewing patterns and which he or she would find relevant based on where he or she resides or works.
Data annotation is tedious and time-consuming. Thankfully, AI (artificial intelligence) systems are now accessible to automate the procedure.
What is a data Annotation tool?
In simple phrases, it is an outlet or a portal that lets experts and specialists annotate label or tag datasets of all categories. It is a medium or a bridge between raw data and the outcomes your machine learning modules would eventually churn out.
Data labeling equipment is a cloud-based or on-prem solution that annotates excellent quality training data for machine learning. While many firms rely on an outer vendor to do complicated annotations, some institutions still have their own equipment that is either custom-built or established on freeware or open-source devices accessible in the market. Such devices are usually constructed to handle particular data types, i.e., video, image, text, audio, etc. The devices offer options or features like bounding polygons or boxes for data annotators to label pictures. They can just choose the option and execute their particular tasks.
What are the Advantages of Data Annotation?
Data annotation is immediately aiding the machine learning algorithm to get equipped with supervised learning procedures for accurate prediction. Nonetheless, there are a few benefits you need to understand so that we can comprehend its significance in the AI world.
Enhances the Accuracy of Output
As much as picture annotated data is utilized for training the machine learning, the precision will be higher. The diversity of data sets used to equip the machine learning algorithm will help understand different characteristics that will help the model operate its database and give adequate results in numerous scenarios.
More Enhanced Knowledge for End-users
Machine learning-based equipped AI models to deliver wholly different and seamless knowledge for end-users. Virtual assistant equipment or chatbots assist the users instantly as per their necessities to solve their questions.
Furthermore, in web search engines such as Google, the machine learning technology provides the most related outcomes using the examination relevance technology to enhance the outcome quality as per the past searching manner of the end-users.
Similarly, in speech recognition technology, virtual assistance is used with the benefit of natural language processes to comprehend human terminology and communication.
Text annotation and NLP annotation are part of data annotation, developing the training data sets to formulate such models delivering more enhanced and user-friendly understanding to various people globally through numerous devices.
Analytics is delivering full-fledged data annotation assistance for AI and machine learning. It is implicated in video, text, and image annotation using all categories of techniques per the consumers' provision. Working with competent annotators to deliver a reasonable quality of training data sets at the lowest cost to AI customers.
Why is Data Annotation Required?
We understand for a fact that computers are competent at providing ultimate outcomes that are not just exact but related and timely as well. Nonetheless, how does an appliance learn to provide such efficiency?
All thanks to data annotation. When machine learning is nonetheless under improvement, they are provided with volume after volume of Artificial Intelligence training data to prepare them better at making judgments and identifying elements or objects.
Only through data annotation could modules distinguish between a dog and a cat, an adjective and a noun, or a sidewalk from a road. Without data annotation, every impression would be the exact same for machines as they do not have any ingrained information or understanding about anything on the planet.
Data annotation is expected to make networks deliver detailed results; help modules specify elements to equip computer speech and vision, and recognize models. For any system or model, data annotation is expected to assure the decisions are relevant and accurate.
What are the fundamental challenges of data annotation?
The expense of annotating data: Data annotation can be done automatically or manually. Nonetheless, manually annotating data compels a lot of effort, and you must also maintain the data's integrity.
Accuracy of annotation: Human omissions can lead to bad data quality and immediately impact the projection of AI/ML models. Gartner’s research highlights that bad data quality costs corporations fifteen percent of their revenue.
Read more about data processing on Nanonets: