Data Matching: A Comprehensive Guide to Ensuring Data Integrity
Businesses produce a lot of data. Around 2.5 quintillion bytes of data every day!
There is a lot of information in the data when used properly. But most of the time, it's riddled with issues like duplicate data, missing data points, and incomplete datasets. Data matching can help businesses overcome some major challenges easily.
Patient data matching is an issue that plagues the healthcare sector, endangering patient safety and costing $6 billion annually. ~Forbes
But this can be solved easily. Using data matching automation platforms, teams can automate data cleaning, mapping, and aggregation before it becomes a problem.
In this article, we will discuss how data matching works, how to automate it in 15 minutes, and the best approaches to data matching. It also mentions the advantages of employing these technologies across many industries.
Want to match data on the go? Use Nanonets to set up automated workflows to match data between databases on autopilot!
What is data matching?
Data matching is the process of finding identical entries from one or more collections of data and unifying the data records. It could be performed between datasets to ensure that data from various datasets is synced.
Potential data redundancies are found by data matching. Which further unites them into one record, known as the "Golden Record." It's the initial practical step throughout most initiatives that call for the fusion of one dataset with another. Besides, when you wish to enhance the accuracy of your database at the access point.
It can eliminate duplicate content or for various data mining applications. Data matching, generally, enables people with significant volumes of data to make more accurate inquiries that yield more accurate results. Many data-matching efforts are made to establish a critical connection between the two large datasets for advertising, safety, or other practical goals.
How does data matching work?
Data matching addresses the issue of determining whether two "entities" are valid and the same.
Each component of the collected data is evaluated and matched to each element of the various databases as part of the procedure, dependent on a data-matching algorithm or programming cycle.
Data linking uses the following two approaches :
- Record linking that is deterministic and relies on numerous matched variables.
- The probability of several IDs matching is the foundation for probabilistic record linking.
The most common data matching method is probabilistic since deterministic linking is too constrained. The data must be arranged or subdivided into equal-sized chunks with matching attributes. The matching conditions are then put in place. For example, identities can be compared using both alphabetic and quantitative matching.
The importance of each attribute is then assessed according to its relative strength. Hence, it is necessary to compute the probability of matching. An algorithm then modifies the relative weights for every parameter.
Want to automate data matching tasks?
Check out Nanonets data automation software. Extract data from any document & perform data tasks on autopilot! Reach out to our teams or set it up yourself.
Why should you use data matching?
When working with massive data, you're bound to have data duplicates. Data matching ensures that your data is reliable, uniform and synched across different platforms.
Data matching improves reliability, effectiveness, and compatibility across various fields and situations. Data matching is one of the first phases in every organization's general data management strategy. You should use data matching to end an organization’s redundant data.
Data Authenticity
Companies use a network of connected apps and data systems. They need to establish a centralized, unified database to use data effectively.
The data matching allows businesses to verify the data and update data records accordingly. For example, suppose an old customer signs a new contract with your company. After invoice data extraction, if you create a new record in Salesforce, it will fudge your numbers. With data matching, you can update the old customer record with new data instead of creating a new entry. This improves data authenticity.
Precision and Effectiveness
Data matching makes it easier to compare, spot similarities, and highlight complex data. It is a dependable instrument that allows for higher accuracy requirements. At the same time, it aids in minimizing irrelevant variables.
Offer Business Insights & Analytics
Data matching can aid data analysis by converting input data to a similar layout. Massive information can be analyzed using analytics software to uncover patterns. But many of these systems demand that customers standardize their data first.
Several workers may enter data, identities, and places into the CRM in various formats. A systems analyst or administrative staff member can use a data-matching technique to change data in many datasets and CRM.
Guarantees Compliance
Many businesses use their datasets to hold compliance data, including agreements with customers and suppliers and permission procedures. Applications for data matching can assist companies in maintaining their datasets. And ensuring that they have adhered to regulatory rules for various accounts.
By identifying identical entities and accounts with similar characteristics. These applications can speed up compliance activities and enhance the productivity of administration workers.
Enriched Data
Data matching integrates an established database with information from reliable third parties to upgrade the organization's data. Businesses can enhance their revenue, advertising, production, and other operations by improving the accuracy and reliability of customer data.
The updated data helps fill in any gaps in the user information. That provides the company with a comprehensive view of its targeted market segments.
Want to automate data matching?
Try Nanonets to match incoming new data with data from different software, csv, googlesheets, etc.
How to match data manually?
Although data matching is a straightforward procedure, there are many moving pieces so it may be stressful. We'll examine a direct four-step approach for matching data records. And include the specifics to which you need to pay attention at each stage to guarantee optimal accuracy.
Step 1: Selection & Preparation Of The Data
During the initial stage, the main focus should be on selecting and collecting the data. Also, you need to identify data quality problems, including blank entries, misspelled words, formatting and sequence variances, etc.
Data must be analyzed, cleansed, and standardized to provide seamless and precise record matching.
i) Data Profiling
By applying statistical methods to existing datasets, data profiling reveals confidential messages about their organization and composition. The quality of your data is highlighted in a dataset profile report. With this data, you may spot chances for database purification. And discover the characteristics that might be players in the recognition process.
ii) Data Standardization
Data standardization is performed to remove the uncertainties discovered in the preceding phase. And provide a consistent perspective throughout all datasets participating in the classification phase.
iii) Choosing Data Attributes
The selection of data characteristics is the last stage in the preprocessing step. You can reduce the output's clutter by choosing the data fields. That you want to maintain for tremendous outcomes or a golden record, choose the required fields, which will be compared to entries to see if they match.
Step 2: Data Match Configuration & Execution
It's essential to configure the matching technique now that your dataset is standardized. And you have chosen matching characteristics. It's vital to note that various techniques offer different settings options.
Though the specifics of these setups may vary depending on the supplier, using them is necessary to guarantee correct results. We highlight five customizable components of the matching procedure below:
i) Analyzing Data From Different Datasets.
You should specify what datasets should match each other in the initial setting. Three comparisons are possible:
a) Within: This option only compares data entries within the same dataset. The first row of Database A will match all other rows of Database A and vice versa. The first row of Database A will be compared to all other rows of Database A and vice versa.
b) Across: This option analyzes relevant data between datasets. For instance, all rows from Dataset A and all from Dataset B would be analyzed.
c) Both: In this arrangement, comparisons are made between and within the linked databases. For instance, Dataset A is matched to Datasets A and B.
ii) Linking Fields From Different Datasets
It is crucial to map sections representing the exact data for analyses conducted between databases. Due to the following differences among various data sources:
a) One resource of data structures, for instance, saves Customer Details as a single field. In contrast, the second resource maintains three domains: First, Middle, and Last Name.
b) Field titles, such as the location column referred to as Residential Address in one resource. At the same time, it is saved as the term Address in another resource.
iii) Find the matching conditions
Just matching data using one field might not be the best idea. It might lead to skewed results. It's best to use multiple fields to match the data points.
Step 3: Assessing the Outcomes
Following the computation of the final scores, you will be provided with the following details. Is a record identical to any other data? How well do the corresponding data match? What are the results of each field's competitive games? You must assess the accuracy of the results after generating them.
- Assessing bogus-positive and false-negative results
- Adjusting data match configuration
Step 4: Merge & Remove Duplicate Data
Eliminating the detected duplication is the final step in the data-matching procedure. There are two methods for getting rid of the duplicates:
- Combine identical records to create a single, comprehensive record
- Choose the complete log to serve as the gold standard, then remove all other duplications.
Both strategies are used to cut duplications and preserve the most data. Additionally, you can create rules that merge and replace data.
If you worry about data verification or data matching, check out Nanonets.
Automate everything from data collection, matching, processing, verification to secure data storage with no-code workflows. Need to know more?
Reach out to our team or start a free trial!
How to automate data matching?
Data matching works on pre-set conditions, which makes it easier to automate with workflows. Data matching automation simplifies data matching and accelerates the pace of data flow and data sync across different data sources.
Let's look at how simple it is to set up data-matching workflows for a use case.
Consider you get an invoice from your vendor. You want to see whether the seller has sent you the proper invoice. You already have their quote details, so you want to match the data before you pay the vendor. Instead of doing this manually, here's how it will look on Nanonets:
Once the invoice file is uploaded to the platform, it will go through particular checks. Here's how extracted invoice data looks like:
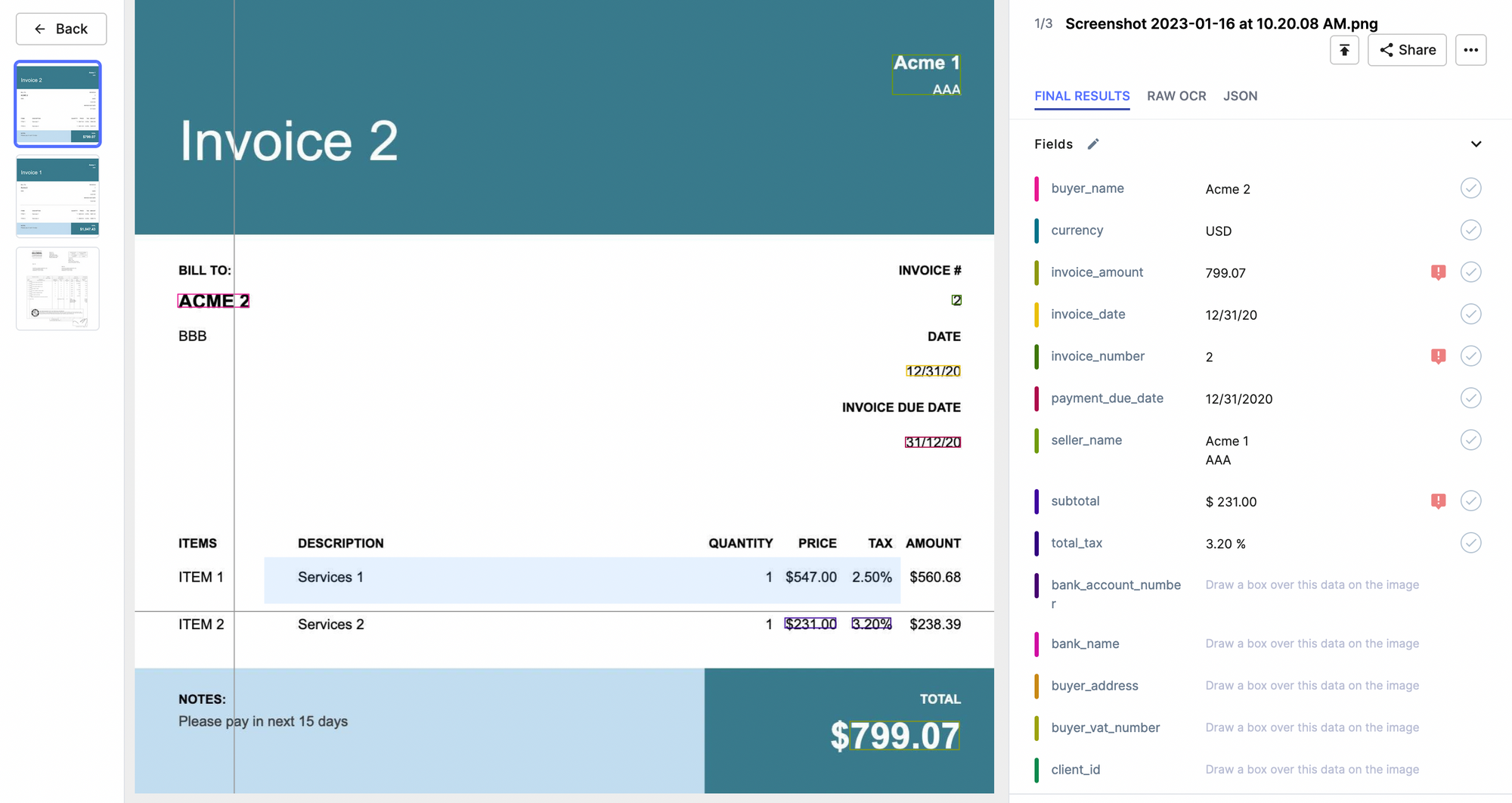
The red flags you see are the data matching issues recognized by the platform. You can set up these data matching conditions easily using workflow conditions:
If there is any error, whatsoever in the file, all the reviewers will get email notifications so they don't miss them:

Once reviewed, the platform updates the required record in the business software of your choice, with over 5000+ integrations.
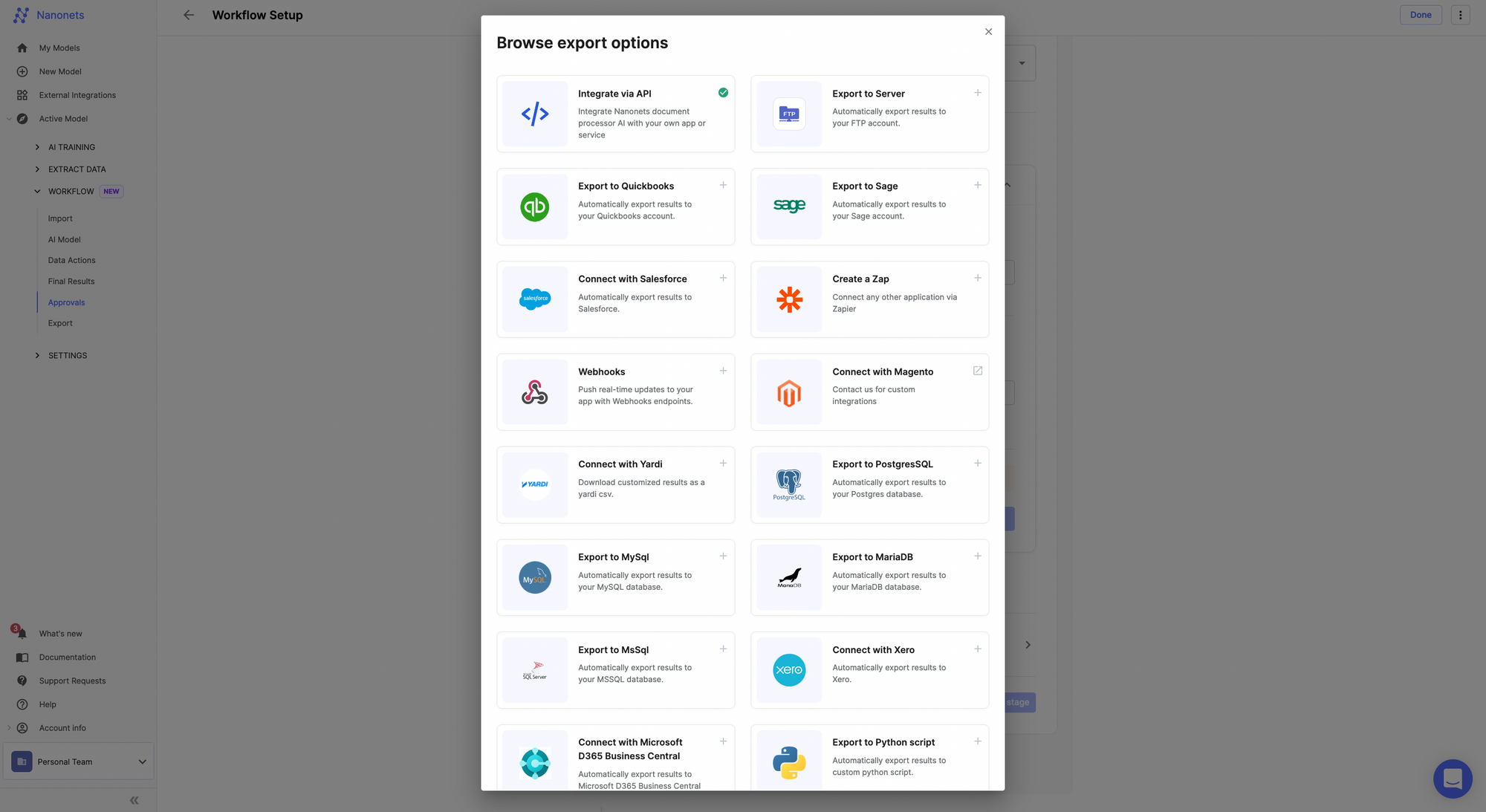
Sounds simple?
It is. Give it a try. Try matching document data with Nanonets workflows. If you need us to set it up for you, set up a call with one of our experts, for free.
Nanonets: Document Data Matching for Enterprises
Every business has a lot of documents. But to use document data effectively, businesses need to use an efficient data automation platform. That;'s where Nanonets come in.
Nanonets is an AI-based OCR software with advanced workflow capabilities. It is the perfect solution for businesses looking to maximize the potential of document data. Whether it is automating document data entry, document verification, document management, or document storage, Nanonets can handle every document-related task efficiently.
Nanonets connects with 5000+ applications, making it easier to quickly match data across platforms, sync data, and validate data points. Nanonets can extract data from any document ( images, PDFs, word documents, CSV, excel) and run automated workflows on extracted data.
Why Nanonets? Well, here are some reasons:
- 7 Day free trial - Explore the platform before you pay
- 24x7 Support
- Free migration assistance - We do the heavy lifting at Nanonets
- A dedicated customer success manager
- Custom pricing plans
Have a usecase in mind? Reach out to our sales team or start your free trial!
Read more:
What are Different Use Cases of Data Matching?
Data matching is the practice of contrasting two collections of existing information. There are many possibilities to achieve efficient data matching. But the procedure is often based on techniques or programmed loops. During this, processors carry out sequential evaluations of each distinct dataset component. Comparing it to a piece of another database or complex variables like strings for resemblances.
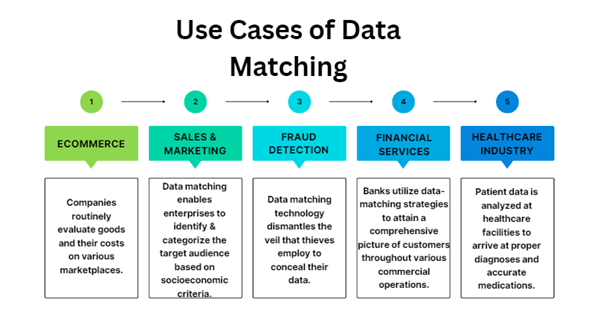
Data matching can be employed for data mining or eliminating redundant data. Many data-matching attempts are conducted for different purposes. Such as to create a crucial connection between two large datasets for marketing, cybersecurity, or practical purposes. Here are typical applications for data matching:
E-Commerce
Companies check goods and their costs on various marketplaces. Even if two items do not share a similar identity or specification, corporate data matching enables the identification and matching of similar products.
Sales & Marketing
The data matching allows enterprises to categorize target audiences based on demographic criteria by merging data optimization and assessment techniques. Yet, creating relevant and fitting advertisements or promotional initiatives for prospective consumers. Personalization enables a business to boost the effectiveness of its promotional activities.
Fraud Detection
By focusing on sections that are going bankrupt and showing suspicious transactions, Data-matching technology dismantles the veil thieves use to conceal their data.
Financial Services
Banks and financial service providers use data matching to complete customer credit ratings. Also, organize projects like finding criminals associated with money laundering. Banks use data-matching strategies to get a comprehensive picture of customers throughout various commercial operations.
Healthcare Industry
Healthcare facilities analyze patients’ data to arrive at proper diagnoses and accurate medications. To ensure the accuracy of patient records, hospitals use data-matching using software solutions.
Suppose the healthcare sector does not use an automated deduplication method. Patients may receive therapy or unsuitable drugs for the same ailment. Health records are linked with various other databases. To investigate the effects of many factors such as treatments, diseases, and medication.
Want to automate repetitive data tasks? Save Time, Effort & Money while enhancing efficiency with Nanonets!
Conclusion
Duplicate removal, comparing, and combining are essential for efficient company operations and intelligence. Businesses have a lower risk of losing out on chances for business growth, client recruitment, improvement of products, and higher revenue. Suppose they resolve redundancies prevalent in their databases. The four stages of the information matching process, preparation, setup or execution, results evaluation, merging, and deduplication, cannot be handled by a single solution.
Find out how Nanonets' use cases can apply to your product.
Read more:
- How to improve data insights with data aggregation?
- How to automate data processing?
- Improve data consistency with efficient data matching
- Turn raw data into structured data with data enrichment
- Find the best data extraction tool in 2023
- Eliminate data inconsistencies with data wrangling
- Automated data processing: Why you needed it yesterday
- Automate mundane data tasks with data automation