AI data entry: How to get started?
It's a sight all too familiar during tax season: the accounting team huddled in a room, heads down, manually sifting through stacks of invoices, expense reports, and receipts, meticulously entering data into spreadsheets. This situation isn't unique to accounting – a Zapier survey revealed that 76% of employees spend up to 3 hours daily on data entry, a massive drain on their talent and potential.
Imagine if your employees could spend that time on meaningful work that moves the needle. AI data entry, powered by machine learning (ML), natural language processing (NLP), and workflow automation, can make that a reality. With robust automated data entry software, organizations have slashed data entry time by up to 95%.
In this article, we'll explore how AI data entry works, explore its key advantages and provide practical tips to ensure a smooth implementation.
What is AI data entry?
AI-powered data entry automates the extraction and input of data into digital systems, eliminating the need for manual typing into forms, applications, or databases. But that's just the beginning.
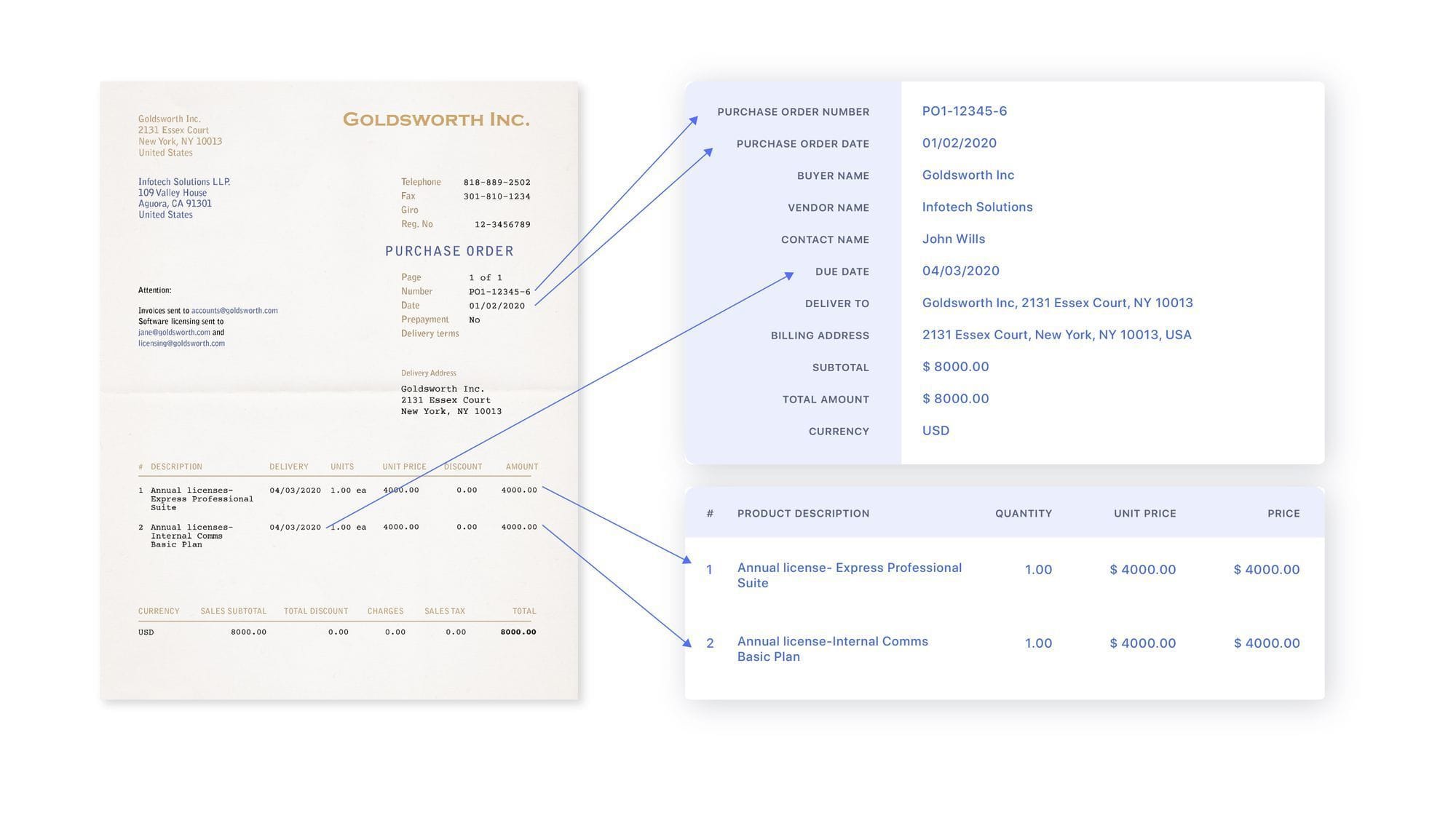
A robust data automation solution can also extract, classify, validate, and export data or auto-populate fields in other software systems, streamlining the entire data handling workflows.
The benefits of AI data entry
A 2020 McKinsey survey revealed that automation slashed costs by 10-15% and dramatically reduced order processing time from 2-3 days to a mere 1-2 hours. While various automated systems contributed to these efficiency gains, AI data entry was crucial in achieving these remarkable results.
AI data entry ROI calculator
Nanonets PRO plan cost = $999/month
In case the number of pages goes beyond 10,000 in a month, an extra fee of $0.1 will be charged for each additional page.
Notes and assumptions (click to expand)
- This ROI calculation focuses solely on data entry-related costs and does not consider the costs of other tools or processes that may be in use.
- The calculation is simplified and excludes additional expenses such as supplies, storage, and potential processing delays.
- This calculation does not reflect the potential for increased revenue from reallocating employee time to higher-value tasks.
- Calculations are based on Nanonets' PRO plan, compared to the cost of manual entry
- The total cost after implementation includes the Nanonets subscription cost, the additional cost per page (if applicable), and the wages of one clerk to manage the system. This assumption may not accurately represent the situation for all businesses, especially larger ones with more complex document processing needs.
- By automating document processing, employees can focus on more meaningful and strategic work, improving job satisfaction and productivity. This benefit is not explicitly quantified in the ROI calculation.
- Consideration of larger ROI benefits from factors not included in this calculation is suggested.
- Nanonets offers a pay-as-you-go model suitable for smaller businesses or lower document volumes, with the first 500 pages free, followed by a charge of $0.3 per page.
Move at light speed with accurate data extraction, faster approval cycles, and seamless data flow between your business systems.
Let’s break down the specific benefits further:
1. Saves cost, time, and effort
Data entry AI tools and other AI technologies could automate up to 62% of current task hours, with the potential to reach 79 and 98 percent by 2030. This frees employees to focus on higher-value work that truly moves the needle.
Moreover, businesses implementing automation have seen a 90% reduction in manual invoice data entry time, demonstrating how automation pays for itself many times over.
2. Improves data accuracy
Accuracy is critical in data entry, whether extracting vital financial figures for a quarterly report or capturing patient symptoms from clinical notes. Even a small error can be costly.
AI tools for data entry, like Nanonets, provide an impressive 95% accuracy rate, seamlessly extracting data from structured and unstructured documents. Terminologies, tax regulations, currency changes, language syntax – all are swiftly recognized and extracted with precision.
3. Enables real-time data flow
Automated data entry systems can directly connect extracted and structured data to other platforms through APIs, ensuring synchronized data across departments. This enhances workflow efficiency, analytics, and reporting, with customer order data automatically pre-populating fields in CRMs, inventory management, and accounting systems.
For instance, invoice approval workflows can be reduced from 5 process steps to 2 by cutting manual handoffs. A scanned supplier invoice received in the accounting email would be categorized, data extracted, posted in the ERP, and approval notification sent – all without manual intervention.
~ Luke Faulkner, Product Manager at Tapi, on how Nanonets has impacted the company.
4. Enables effortless scalability
AI data entry solutions can process thousands of documents daily without losing steam, whether from an email inbox, a shared folder, website form submissions, or scanned copies. The ability to scale infinitely is a powerful advantage.
Moreover, these intelligent systems learn from each use, becoming more refined and accurate over time – a game-changer for data-intensive industries like healthcare and finance.
How to implement AI data entry
Manually entering data into spreadsheets is about as thrilling as watching grass grow – it's mind-numbingly tedious, especially when it's a day-in, day-out task. The monotony not only dulls the senses but also makes you more prone to errors. And this isn't an isolated issue. A recent study by EY revealed that payroll employees spend almost 29 weeks a year correcting the most common payroll errors.
Thankfully, now AI is advanced enough to automate capturing, classifying, and structuring data; it can optimize workflows and unlock major efficiency improvements. Here's a step-by-step guide to help you successfully implement and use AI for data entry:
1. Identify suitable processes for automation
Assess your current data entry workflows and pinpoint time-consuming, repetitive, rule-based processes that are prone to errors. Prioritize those with the highest impact on your business and most suitable for automation, such as invoicing, form processing, or customer profile updates.
2. Define your goals and requirements
Establish specific, measurable goals that align with your business needs and determine the required level of accuracy, processing speed, and scalability. The goal could be reducing costs, improving accuracy, or streamlining workflows.
3. Choose the right AI data entry solution
Research and compare different AI data entry solutions available in the market. Look for solutions that offer:
☑️ Integrates with your tech stack
- Does the solution allow easy integration with your existing IT infrastructure through APIs?
- Can it export data directly into your databases, ERPs, or other platforms?
☑️ Accuracy and effectiveness
- Does it leverage advanced AI and machine learning for data extraction?
- What accuracy rates does it achieve in testing based on your sample documents?
- Does it provide transparency around confidence scores?
☑️ Customization ability
- Can you train custom models tailored to your unique document templates?
- Does it allow configuring specific data fields for capture as per your needs?
☑️ Scales up and down
- Can it handle spikes in your document volumes without losing accuracy?
- How quickly can documents be processed without latency issues?
☑️ Secures your sensitive data
- Does the vendor provide enterprise-grade data security?
- Can your sensitive business data be encrypted in transit and at rest?
☑️ Pricing and support
- Is pricing aligned with the value derived from automation?
- Is responsive customer support available across implementation cycles?
We may be biased, but we believe Nanonets' AI-based Intelligent Document Processing platform checks all these boxes.
With cutting-edge OCR, pre-trained and custom AI models, seamless integrations, top-notch security, and an intuitive interface, Nanonets makes automating data entry a breeze. You get unparalleled accuracy, lightning-fast turnaround times, and the ability to extract data from a vast array of documents, supporting over 100 languages and various file formats.
Moreover, with direct integrations, API access, Webhooks support, and a Zapier connector, you can automatically export the extracted data to your ERP, CRM, accounting software, or any other business application.
Data security and compliance are top priorities for Nanonets. The platform adheres to strict security standards like SOC 2 and complies with major regulations like GDPR and CCPA. Your data is encrypted, and you will have control over user access and permissions.
Nanonets offers a comprehensive suite of features to streamline your data entry workflows, including automated document import, intelligent sorting and routing, data validation, and matching master records. With flexible options for pre-trained and custom AI models, Nanonets can scale to meet your specific requirements, processing millions of documents per month while prioritizing data security and compliance.
4. Prepare your data and infrastructure
Clean and preprocess your existing data, remove duplicates, correct errors, and standardize formats. Organize your documents and data sources in a structured manner and set up the necessary infrastructure to support the AI data entry solution.
5. Train and validate the AI model
Train your AI model using a diverse dataset representing various document types and formats. Provide labeled examples to help the model accurately extract and classify data. Regularly validate the model's output and fine-tune its performance using active learning and human feedback loops.
6. Implement and integrate
Integrate the AI data entry solution into your existing workflows and systems, ensuring smooth data flow between the AI model and downstream applications. Set up automatic data validation and error handling mechanisms and establish clear processes for human intervention when needed.
7. Monitor and optimize
Continuously monitor the performance of your AI data entry solution, tracking key metrics like accuracy rates, processing times, and error rates. Identify areas for improvement, regularly update the AI model with new training data, and optimize your workflows based on insights gained from the AI system and user feedback.
These steps can help you successfully implement AI data entry in your organization, revolutionizing your data entry processes and unlocking significant efficiency gains.
AI data entry real-world applications
AI data entry is being adopted across various sectors, including finance, healthcare, insurance, and logistics.
Let's explore some real-world applications of AI data entry across various industries and see how it's transforming the way businesses operate.
1. Process invoices, bills, receipts
Automating the processing of documents like invoices, bills, and receipts can save countless hours for your workforce. Robust solutions can take care of the process end-to-end, with you just overseeing final accuracy and authorizing payments.
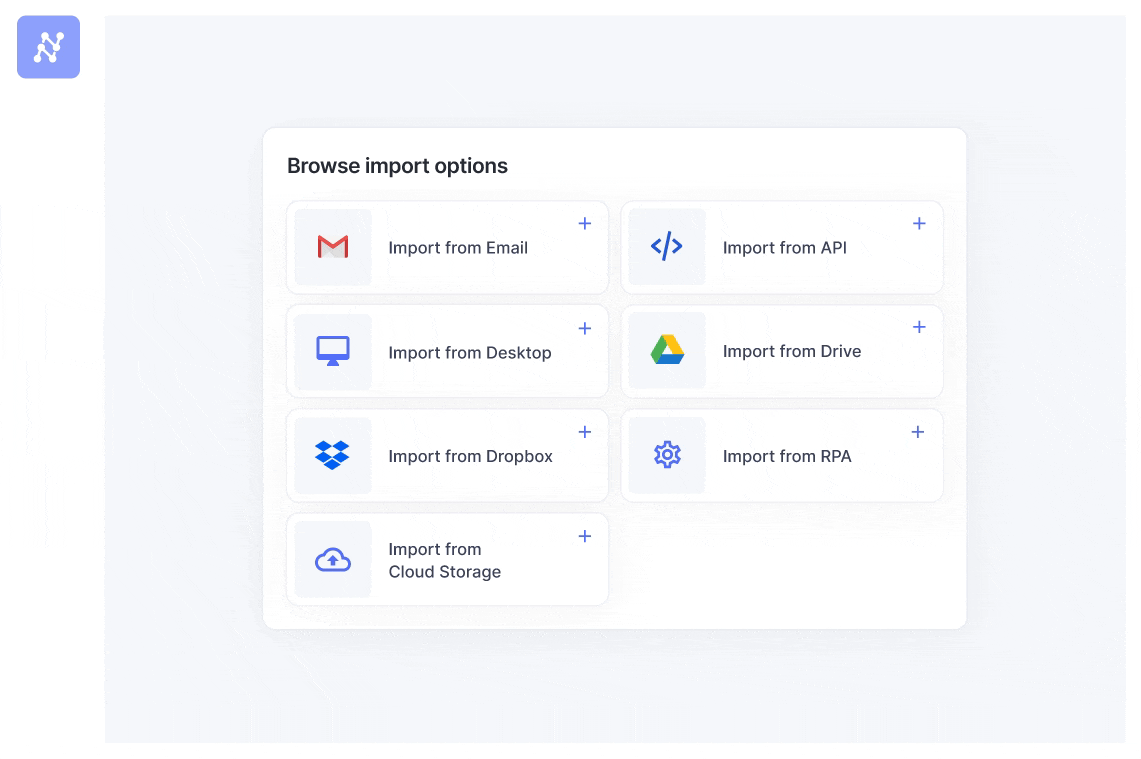
With Nanonets, all you’ve to do is select an AI model that suits your documents and then set up an auto-import option from wherever they are coming in — your email inbox, a cloud storage folder, or even a physical scanner. If you only have a handful of documents, you can upload them manually.
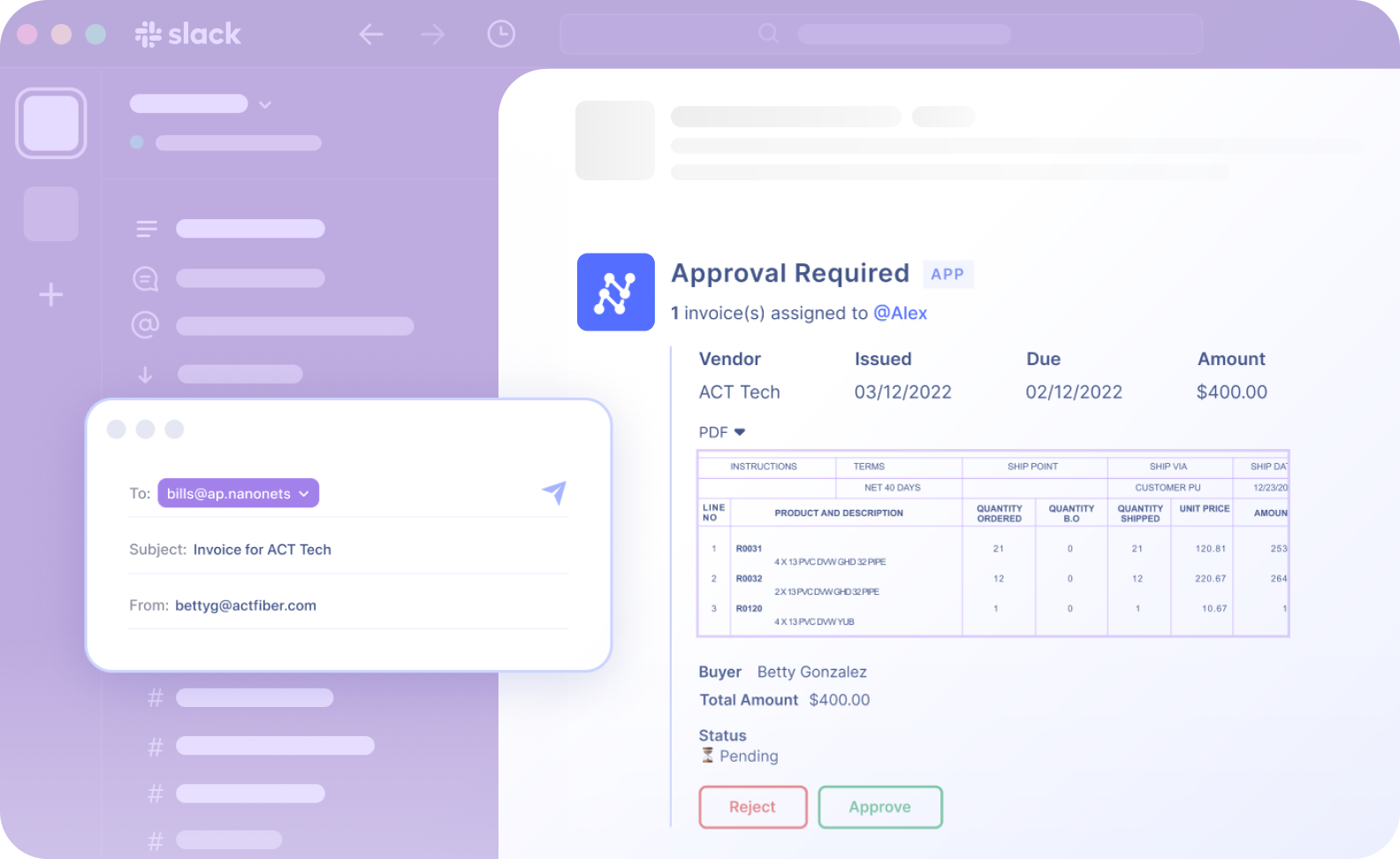
After that, the AI captures the information, validates it, and sends it for approval. You can then review and approve the data in a central hub. You can export it as an Excel file or directly send it to your accounting software, ERP, or other systems.
2. Turn form submissions into actionable data
If you’re running a small designer boutique, you may collect orders via the Typeform link embedded in your Instagram bio or via a form on your Webflow website.
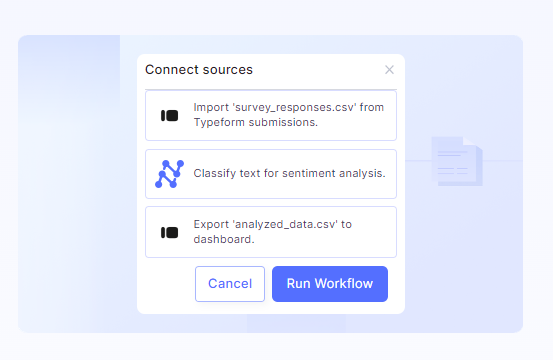
With Nanonets, these form submissions can be turned into actionable data. The Zapier integration allows you to extract and push everything directly from the customer's name, contact information, product choice, style preferences, and delivery details into your CRM, sales, or delivery management software.
Sales orders can be immediately picked up. The specifics of each order will be noted and delivered to your inventory management system. It allows you to provide a more personalized customer experience, streamlining operations and improving responsiveness.
3. Automate customer profile updates
Profile data is critical, whether it is a patient’s medical history in a hospital or a customer’s driving record in a car rental company. You can’t expect the customer or a patient to fill out long, tedious forms each time.
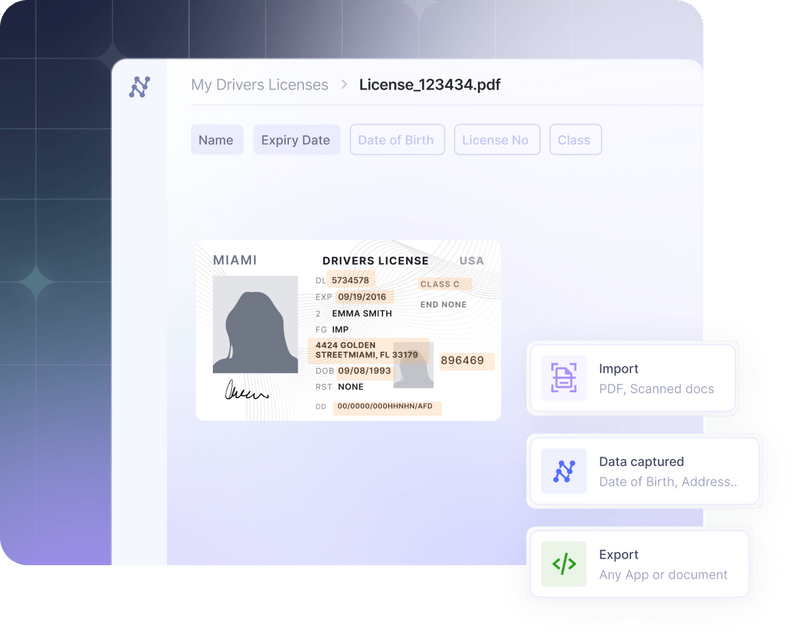
Utilizing Nanonets, you can scan documents they submit — new test reports and driving licenses — and immediately update the profile data without involving frontline staff. The AI can extract data from these documents and classify and update the information directly in your CRM, EMR, or proprietary software.
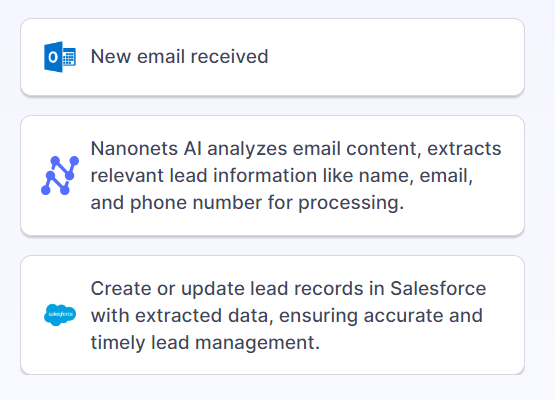
This saves your customers from the frustration of constantly filling out forms and your staff from mind-numbing data entry tasks. It also ensures that data stays updated and always available, helping you provide better customer experiences.
4. Manage vendor and supply chain documentation
Supply chain and vendor management often involve dealing with large amounts of documentation: purchase orders, quotations, invoices, contracts, delivery receipts, and more. Nanonets can automate the extraction and classification of data from these complex documents.
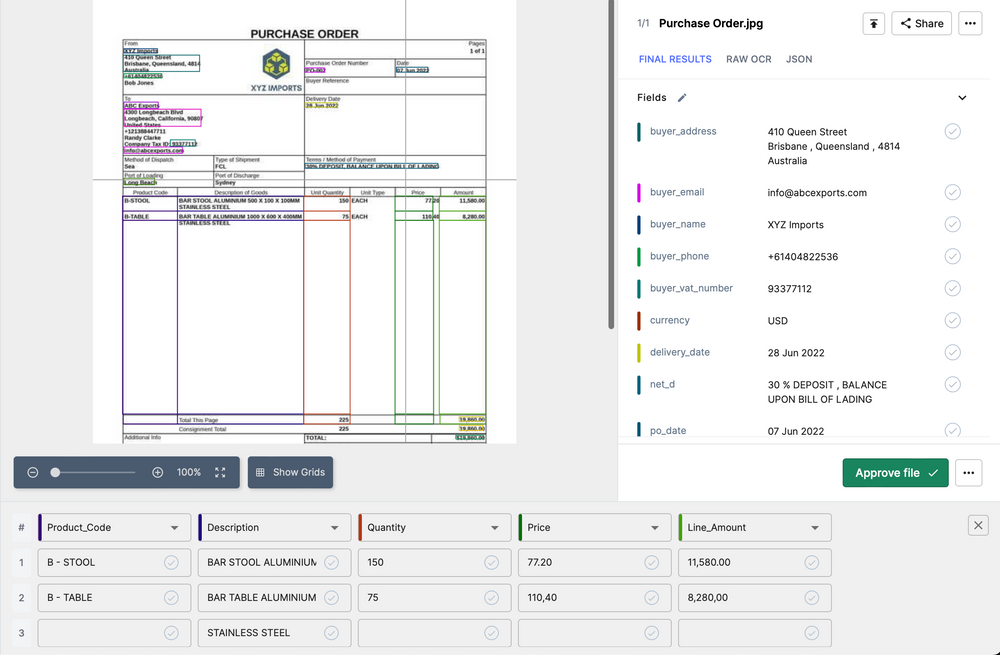
Purchase orders can be scanned to capture line item descriptions, pricing, delivery schedules, and more. Currency amounts, tax details, and payment deadlines can be pulled from complex vendor invoices. Delivery receipts can reveal shipment contents and tracking IDs for logistics visibility.
It can also interpret document formats, currency types, languages, and legal terminologies. Also, Nanonets allows documents to be processed as soon as they arrive — ensuring timely updates even during holidays, late nights, or other non-business hours.
5. Optimize parsing and verification workflows
HR and marketing teams often grapple with processing large volumes of data from sources like resumes, feedback surveys, and campaign analytics. AI tools like Nanonets can automatically parse these unstructured datasets to extract the required details.
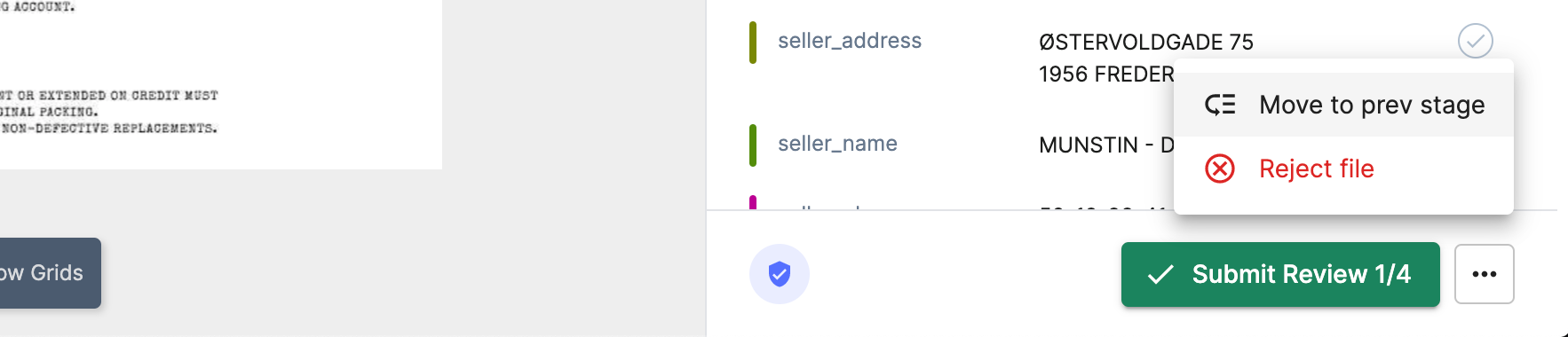
For HR, it could mean capturing skills and experience information from resumes or validating details from identity documents. For marketing teams, it could capture customer sentiment from surveys or lead capture data that could be handed over to the sales team.
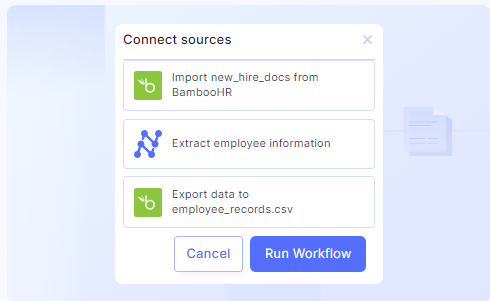
The extracted data can flow seamlessly into ATS, CRMs, or marketing automation platforms. This eliminates tedious manual efforts, minimizes errors, and allows employees to focus on strategic analysis.
6. Streamline expense report processing
Processing expense reports can be a time-consuming task for finance teams, especially in large organizations with numerous employees. With Nanonets, you can automate the extraction of data from receipts, invoices, and other expense-related documents.
The AI can capture details like date, vendor name, amount, and expense category, and automatically populate expense reports. This reduces manual data entry, minimizes errors, and ensures faster reimbursement processing. The data can also be seamlessly integrated with your accounting software for easier reconciliation and reporting.
7. Automate data entry for legal contracts
Legal teams often deal with a large volume of contracts, agreements, and other legal documents. Nanonets can help automate the data entry process for these documents, extracting key information such as party names, dates, clauses, and obligations.
The extracted data can be used to populate contract management systems, making it easier to track and manage legal agreements. This not only saves time but also reduces the risk of manual errors and ensures that important contractual obligations are not missed.
8. Simplify insurance claims processing
Insurance companies receive a large number of claims daily, each accompanied by various supporting documents such as claim forms, medical reports, and bills. Nanonets can automate the data entry process for these documents, extracting relevant information like policyholder details, claim amounts, and medical codes.
The extracted data can be automatically populated into the claims management system, reducing manual effort and speeding up the claims processing cycle. This leads to faster claim settlements and improved customer satisfaction.
9. Automate data capture for logistics and shipping
Logistics and shipping companies handle a large volume of documents such as shipping labels, bills of lading, and customs forms. Nanonets can automate the data capture process for these documents, extracting key information like shipping addresses, tracking numbers, and product details.
The extracted data can be automatically updated in the logistics management system, enabling real-time tracking and faster processing of shipments. This improves operational efficiency and reduces the risk of delays or errors in the shipping process.
These are just a few more examples of how AI data entry can be applied across various industries and business functions. The possibilities are endless, and with a powerful tool like Nanonets, organizations can automate their data entry processes, saving time, reducing costs, and improving accuracy.
Final thoughts
The days of manual data entry are over. Modern automation solutions present a great chance to hand over the drudgery to AI. Now, your team can focus on meaningful work that moves the needle.
With Nanonets, you can automate repetitive data entry tasks with accuracy. And thanks to seamless integrations, data flows directly between your systems in real time. This means happier employees, delighted customers, and a competitive edge. Try out Nanonets today for free.
FAQs
Is there AI for data entry?
Yes, Nanonets leverages AI-powered optical character recognition (OCR) to automate data extraction from documents. For example, Nanonets can scan supplier invoices and automatically pull vendor names, amounts due, and payment terms into a structured format. This eliminates manually typing the data into your accounting system.
Will data entry be replaced by AI?
AI data entry solutions like Nanonets will significantly reduce the need for manual data entry but may not completely replace humans. Humans will still be needed to train AI models, validate outputs, handle exceptions, and make judgment calls.
Can you automate data entry?
Absolutely. With Nanonets, you can build no-code automation to extract data from documents and webpages and then flow it directly into databases, Excel, ERP platforms, etc. For instance, scanned delivery receipts can automatically capture shipment details like tracking IDs, contents, and dates into inventory management software.
How do I automate data entry in Excel?
AI-powered automation tools like Nanonets can seamlessly integrate with Excel, allowing you to populate spreadsheets with extracted data automatically. For example, customer feedback captured in Typeform surveys can be automatically structured and pushed into Excel -- no manual copying-pasting is needed. Just set up Nanonets, build automation workflows, and export data in one click.